ALL >> Education >> View Article
Integrated Machine Learning And Ai In Biological Research
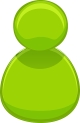
Advancements in DNA sequencing, driven by machine learning and AI in biological research, have dramatically accelerated the process. Sequencing the human genome now takes a single day, a feat that previously required a decade using traditional methods.
According to Markets and Markets, the global artificial intelligence market in healthcare is forecasted to reach an impressive US$4.54 billion by 2029.
Are you interested in becoming a part of this transformative field of bioinformatics?
This blog post briefly introduces how machine learning and artificial intelligence revolutionizing biological research, leading to unprecedented discoveries and accelerating scientific progress.
Integration of Machine Learning and AI in Biological Research
According to a report from BMC Medicine, integrating ML and AI techniques in life-course epidemiology offers remarkable advancement opportunities. It is to understand the complexity between biological, environmental, and social factors shaping health trajectories across the life cycle.
Machine learning and artificial intelligence technologies have ...
... been increasingly applicable in epidemiological studies. It demonstrates the ability of Machine Learning and AI in Biological Research to handle complex datasets, identification of intricate patterns and associations, integrate multimodal data types, enhance casual interface methods, and improve predictive models.
Applications of Artificial Intelligence in Biology
Data Analysis and Interpretation
Bioinformatics
AI algorithms are revolutionizing the analysis of massive artificial intelligence in biological datasets, offering unprecedented insights into the complexities of life. AI in bioinformatics excels at identifying intricate patterns within the vast expanse of genetic information in genomics. Machine learning models can analyze DNA sequences to pinpoint genetic variations associated with diseases, predict disease risk, and even personalize treatment plans.
Drug Discovery
Artificial Intelligence is revolutionizing drug discovery by accelerating several key stages of the process. One significant way AI in bioinformatics contributes is by identifying potential drug targets. The algorithms can analyze vast amounts of biological data, such as genetic sequences, protein structures, and disease pathways, to pinpoint cellular processes or molecules that could be effectively targeted by drugs. This helps researchers focus their efforts on the most promising avenues for drug development.
Personalized Medicine
AI empowers personalized medicine by analyzing individual patient data, including genomics and medical history. This analysis allows AI to predict disease risks, identify optimal treatment options, and tailor treatment plans for each patient. By considering unique factors like genetic predispositions and individual responses to medications, AI helps healthcare providers make more informed decisions, leading to improved treatment outcomes, reduced side effects, and ultimately, better patient care.
Image and Signal Processing
Medical Imaging
According to a report from Oxford Academic Journal, medical imaging analysis involves using advanced techniques to examine X-rays, MRIs, and other scans. This allows for the detection of diseases like cancer or heart conditions, monitoring their progression over time, and providing crucial guidance for surgical procedures presented by the National Library of Medicine. By analyzing these images, healthcare providers or professionals can make more informed decisions about patient care, leading to improved outcomes and potentially saving lives.
Microscopy
Microscopic image analysis involves sophisticated techniques to examine cellular structures and activities. By employing advanced algorithms, researchers can automatically identify individual cells within an image, categorize them based on their morphological characteristics (size, shape, texture), and monitor dynamic processes like cell division, migration, and interactions. This automated analysis significantly accelerates research in various fields, including biology, medicine, and materials science, by providing rapid and objective insights into cellular behavior.
Biological Modelling and Simulation
Systems Biology
Systems Biology is a field that aims to understand the intricate workings of biological systems by creating and simulating complex models, such as the human immune system (Bioinformatics Advanced). The system biology models incorporate various components, including cells, molecules, and their interactions, to predict how the system behaves under different conditions. By simulating the immune system’s response to various stimuli, including infections, vaccines, and diseases, researchers can gain valuable insights into its function and identify potential targets for therapeutic interventions.
These approaches to applications of artificial intelligence in biology can accelerate drug discovery, personalize treatments, and ultimately improve human health.
Artificial Intelligence in Biological Data
Handling Big Data
Modern biological experiments, such as high-throughput sequencing and proteomics, generate massive and complex datasets that are beyond the capacity of traditional data analysis methods. Artificial Intelligence in Biological Data plays a crucial role in handling this data deluge. AI algorithms, particularly deep learning models, excel at processing and analyzing large volumes of data, identifying intricate patterns, and extracting meaningful information. This enables researchers to gain deeper insights into biological systems and accelerate the pace of scientific discovery.
Data Mining and Knowledge Discovery
Artificial Intelligence in Biological Data empowers researchers to effectively mine vast biological databases for valuable knowledge. AI algorithms, such as machine learning and data mining techniques, can identify hidden patterns, relationships, and anomalies within these datasets. This allows for the discovery of novel drug targets, the identification of biomarkers for diseases, and a better understanding of complex biological processes. By uncovering these hidden insights, Artificial Intelligence in Biological Data accelerates the development of new therapies and improves our understanding of life itself.
Predictive Modelling
Machine learning and AI in Biological research data enable the development of powerful predictive models that can forecast biological phenomena with unprecedented accuracy. These models can predict disease outbreaks, assess individual patient responses to drugs, and even model evolutionary trajectories. This predictive power has significant implications for personalized medicine, public health, and environmental conservation. By leveraging the capabilities of Artificial Intelligence in Biological Data, researchers can anticipate future events and develop proactive strategies to address critical challenges in healthcare and beyond.
Artificial Intelligence and Bioinformatics
Artificial Intelligence and Bioinformatics
Bioinformatics serves as a crucial driver of artificial intelligence in biological data by providing the essential computational infrastructure and analytical tools. It generates massive datasets from biological experiments like genomics, proteomics, and metabolomics. Often complex and high-dimensional datasets require sophisticated computational methods for analysis and interpretation. Artificial intelligence and bioinformatics tools enable the extraction of meaningful information from this data, such as identifying patterns, predicting structures, and understanding biological processes. This curated and organized data then becomes the fuel for AI algorithms, allowing them to learn complex relationships, make accurate predictions, and ultimately accelerate biological discovery.
Moreover, AI algorithms are significantly improving the accuracy and speed of sequence alignment and analysis, enabling researchers to rapidly identify homologous genes and understand evolutionary relationships.
AI-powered methods are enabling more sophisticated gene prediction and annotation, leading to a more comprehensive understanding of the genetic code and its function. Perhaps most significantly, it enables the seamless integration of machine learning and artificial intelligence in biological research with diverse data types, such as genomics, proteomics, and metabolomics. This approach provides a more holistic view of biological systems, allowing researchers to uncover complex interactions and gain deeper insights into disease mechanisms and drug development.
Challenges and Future Directions
Data Quality and Availability
One of the major hurdles in leveraging machine learning and AI in biological research is the quality and accessibility of biological data. Biological datasets are often characterized by significant heterogeneity, noise, and missing values. Inconsistent data collection methods, experimental variations, and the complexity of biological systems contribute to these issues.
Moreover, access to high-quality data can be restricted due to privacy concerns, proprietary interests, and limited data-sharing practices. Addressing these challenges requires robust data curation efforts, standardized data formats, and the development of innovative data integration and cleaning techniques.
Ethical Considerations
The ethical implications of machine learning and AI in biological research are profound. Concerns surrounding data privacy and security are paramount, especially when dealing with sensitive personal and genetic information algorithms can inadvertently perpetuate existing biases present in the training data, leading to unfair or discriminatory outcomes in areas such as drug discovery and personalized medicine.
Furthermore, the responsible use of AI-powered technologies, such as gene editing tools, requires careful consideration of societal and ethical implications. Establishing clear ethical guidelines and frameworks for AI research in biology is crucial to ensure that these technologies are developed and deployed responsibly.
Interdisciplinary Collaboration
The successful integration of machine learning and AI in biological research necessitates strong interdisciplinary collaboration. Biologists possess in-depth knowledge of biological systems and experimental design, while computer scientists and engineers bring expertise in AI algorithms, data science, and computational methods.
By fostering collaborative research environments, researchers can leverage the strengths of each discipline to address complex biological questions and develop innovative solutions. This interdisciplinary approach will be crucial for overcoming the challenges associated with data quality, ethical considerations, and the development of robust AI-powered tools for biological discovery.
Conclusion
Artificial intelligence and bioinformatics are revolutionizing biological research, offering unprecedented capabilities to analyze complex data, identify patterns, and accelerate discoveries. Its algorithms can sift through massive datasets of genomics, proteomic, and other biological information, uncovering hidden connections, and insights that would be impossible for human researchers to detect alone. This has led to significant advancements in areas such as drug discovery, personalized medicine, and the understanding of fundamental biological processes.
In drug development, artificial intelligence and bioinformatics are accelerating the identification and new therapeautic development process. Its algorithms can predict the efficacy and safety of potential drug candidates, and optimize drug delivery and cost of bringing new medications to the market.
Personalized medicine aims to tailor treatments to individuals based on their unique genetic makeup and other factors that are significantly impactful. By analyzing a patient’s genetic data, medical history, and lifestyle factors to predict their risk of developing certain diseases and to recommend the most effective treatment options. Applying this approach holds the promise of more effective and targeted treatments with fewer side effects.
Moreover, AI in Bioinformatics is driving ground-breaking discoveries in our understanding of fundamental biological processes. Artificial intelligence-powered tools are enabling researchers to analyze complex biological systems, such as the human brain and the immune system, at an unprecedented level of detail. This may lead to new insights into the mechanisms of disease, aging, and other fundamental biological processes that pave the way for new therapies and interventions.
The future of artificial intelligence and bioinformatics is incredibly promising. As AI technologies continue to advance, we can expect to see even more transformative breakthroughs in areas such as personalized medicine, drug development, and our understanding of fundamental biological processes. The integration of machine learning and AI in biological research is accelerating scientific discovery and holds the potential to revolutionize healthcare and improve human health on a global scale.
In conclusion, you have the opportunity to learn more in the bioinformatics and artificial intelligence & machine learning course. CliniLaunch offers a one-stop solution for aspirants who want to train educate and grow their career in the field of healthcare. To learn more, visit: https://clinilaunchresearch.in/best-bioinformatics-courses/.
Add Comment
Education Articles
1. How Exercise Books Foster Organization And CreativityAuthor: The School Print Company
2. Diy Vs. Professional: Choosing The Right Approach For Your School Logo
Author: The School Print Company
3. Top-rated Icse High School In Bhopal For Quality Education
Author: Ronit Sharma
4. Best B. Tech And Engineering College In Meerut And Up
Author: CONTENT EDITOR FOR SAMPHIRE IT SOLUTIONS PVT LTD
5. The Five Borough Academic: Cross-cultural Research In Nyc's Diverse Communities
Author: jonesmiller
6. The Future Of Advertising: How Cgi Is Replacing Traditional Commercials
Author: Rajat Sancheti
7. What Is Iso 27001 Procedures And Why Do They Matter?
Author: john
8. Devops Foundation Certification Course
Author: Simpliaxissolutions
9. The Best Gcp Devops Online Training Institute In Bangalore
Author: visualpath
10. Best Hotel Management College In Delhi
Author: harsh thapa
11. Servicenow Online Training | Servicenow Course In India
Author: krishna
12. Best Google Cloud Platform Ai Training In Hyderabad
Author: visualpath
13. Aws Data Engineering Online Training | Data Analytics
Author: naveen
14. Oracle Fusion Cloud Hcm | Oracle Fusion Hcm Training In Pune
Author: visualpath
15. Why Choose The Certified Big Data Foundation? Key Certification Objectives Explained
Author: gsdc