ALL >> Education >> View Article
The Importance Of Data Cleaning In Data Science
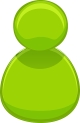
With big data, in an era where organizations are very dependent on data-driven decisions, the quality of data has become the most critical factor. Data cleaning, often called data preprocessing or data scrubbing, is one of the crucial steps in the data science workflow. Without clean, accurate, and reliable data, even the most advanced algorithms and analytical tools can lead to misleading results.
Mastering data cleaning is a basic skill for aspiring data scientists. A data science course training or Data Science course in Hyderabad can expose you to hands-on data cleaning techniques and their practical applications, preparing you to face real-world challenges.
What is Data Cleaning?
Data cleaning is the process of finding, correcting, or eliminating errors, inconsistencies, and inaccuracies in datasets. These kinds of error can include missing values, duplicate records, outliers, or incorrect entries of data. It focuses on making sure that data is complete, accurate, and ready to be analyzed.
Clean data forms the basis of sound analytics, good machine learning models, and business insights. This ...
... step is thus skipped at the peril of invalidating the reliability of results and subsequently flawed decisions, which may result in devastating implications for an organization.
Why Is Data Cleaning Important?
1. Improves Data Accuracy
Errors or inconsistencies in data can distort the results of analysis. Through cleaning the data, organizations ensure that their insights are based on accurate and trustworthy information, which helps them make better decisions.
For example, if there are duplicate entries in customer data, then it may overestimate the size of the customer base or misallocate resources.
2. Improves Model Performance
The sensitivity of machine learning models to the quality of input data is very high. Clean data improves the accuracy of the model, reduces bias, and helps the model generalize well to new, unseen data.
Outliers, missing values, or irrelevant features can hurt the performance of the model. Data cleaning resolves these issues and makes the data more suitable for training and evaluation.
3. Saves Time and Resources
Unclean data can cause delays and inefficiencies in data analysis and model development. Data scientists often spend a significant portion of their time cleaning and preparing data before diving into analysis or modeling.
Organizations can save valuable time and resources by prioritizing data cleaning, streamlining workflows, and increasing productivity.
4. Supports Regulatory Compliance
In finance and healthcare, clean and accurate data is a requirement since regulatory compliance is critical to industries. For example, GDPR and HIPAA are regulations whose requirements must be met by thorough cleaning and validation of data.
Data quality is the reason that ignoring it might result in financial loss, legal penalties, and reputational damage.
5. Enabling Effective Decision-Making
Clean data offers a solid basis for actionable insights. Decision-makers depend on such insights to determine opportunities, risks, and the need to optimize strategies.
For example, retail companies use clean sales data to analyze purchasing trends and design targeted marketing campaigns, maximizing ROI.
Common Challenges in Data Cleaning
Despite its importance, data cleaning can be a complex and time-consuming process. Some common challenges include:
Missing Data: Gaps in datasets due to incomplete surveys, system errors, or data corruption.
Inconsistent Data: Variations in data entry formats, such as inconsistent date formats or naming conventions.
Outliers: Extreme or unusual values that can skew analysis.
Duplicate Entries: Redundant data points that can lead to inflated results.
Irrelevant Features: Attributes that do not contribute to the analysis or modeling goals.
Learning to address these challenges is a vital aspect of any data science course training.
Key Steps in Data Cleaning
1. Identify and Understand the Data
Begin by exploring the dataset to understand its structure, features, and potential issues. Tools like descriptive statistics, visualizations, and summary reports help in identifying anomalies or inconsistencies.
2. Handle Missing Values
Determine the cause and impact of missing data. Depending on the scenario, you can address missing values by:
Removing incomplete records.
Imputing missing values with mean, median, or mode.
Using advanced techniques like predictive modeling for imputation.
3. Remove Duplicates
Identify and eliminate duplicate entries to ensure data integrity. This step is crucial for maintaining accurate analyses and preventing biased results.
4. Resolve Inconsistencies
Standardize formats for dates, units, or categorical variables to ensure uniformity across the dataset.
5. Handle Outliers
Detect and analyze outliers to determine if they are errors or valid observations. Based on their impact, decide whether to retain, transform, or remove them.
6. Validate Data
Cross-check the cleaned data with source systems, apply validation rules, and run test analyses to ensure that the cleaned data meets the quality standards.
Tools for Data Cleaning
There are several tools and libraries that make data cleaning easier, such as:
Python: Libraries like Pandas, NumPy, and OpenRefine are popularly used for cleaning and preprocessing data.
R: Provides strong data manipulation packages like dplyr and tidyr.
Excel: Useful for small datasets with built-in cleaning functions.
ETL Tools such as Talend and Informatica automate the tasks of extraction, transformation, and loading.
This is the level of practice by which the one who joins in Hyderabad Data Science course can actually get to his or her objectives.
The Application of Data Cleansing in Data Science Ecosystem of Hyderabad:
Hyderabad, a city now becoming the nerve center for IT and technology, has emerged as a center of excellence for learning data science, innovation, and development. Most of the world's leading organizations rely on data-driven strategies and, therefore, seek highly skilled data scientists who specialize in data cleaning.
A Data Science course in Hyderabad will prepare the learner to face real-world data challenges by exposing him to cutting-edge tools and techniques. Professionals will be able to ensure that their analyses are accurate, reliable, and impactful with a strong foundation in data cleaning.
Conclusion
Data cleaning forms a crucial process in the process of data science. It provides assurance that any analyses and models will be well based on the quality of data. Businesses derive meaningful insights through addressing errors, inconsistencies, and inaccuracies while making informed decisions, driving growth forward.
Mastering data cleaning is not just a skill but a necessity for aspiring data scientists. A data science course training or a Data Science course in Hyderabad provides the ideal opportunity to get hands-on experience in this critical area. Clean data is your foundation, and it unlocks the true potential of analytics and contributes to the success of data-driven organizations.
For more information visit our website:-
https://bostoninstituteofanalytics.org/data-science-and-artificial-intelligence/
Add Comment
Education Articles
1. Key Features To Look For In An Online Ib Tutor For Academic SuccessAuthor: IB Tutor
2. Calcutta University Distance Degree Programs | Fees, Admission
Author: Studyjagat
3. Data Science With Generative Ai Online Training
Author: Hari
4. Best Google Cloud Ai Training In Bangalore | Visualpath
Author: visualpath
5. Ai Security Online Training In Bangalore | Ai Security
Author: gollakalyan
6. Mlops Training In Hyderabad | Mlops Course In Ameerpet
Author: visualpath
7. Salesforce Training Institute In Hyderabad | Visualpath
Author: Visualpath
8. Prompt Engineering Course | Prompt Engineering Ai Course Online
Author: Susheel
9. Scrum Master Certification | Scrum Master Course In India
Author: visualpath
10. Mendix Training In Chennai | Mendix Online Training
Author: himaram
11. Best D365 Project Management Accounting Training In Chennai
Author: Pravin
12. Aws Data Engineering Training In Bangalore | Aws Data Analytics Training
Author: naveen
13. Read With Ease: How Meditation Helps You Absorb More
Author: Harry
14. What Are The Benefits Of Implementing Iso 29993 In Training Provider Organizations?
Author: john
15. Best Ngo In Delhi: Transforming Lives With Dayitwa Ngo
Author: Elina Gilbert