ALL >> Health >> View Article
4 Ways To Improve Clinical Data Quality In The Digital Era
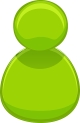
The transition from paper to electronic data capture (EDC) in the clinical trial environment caused a shift in how we look at clinical data management (CDM) quality metrics. The paper world understood that the quality of clinical data obtained was just the quality of the transcription job teams did when transferring data from paper to a database.
The paper versus database Quality Control (QC) had a predetermined criterion for sampling of N+1 or 20 individuals, whichever was smaller, and a 100% QC of essential variables.
Acceptable error rates were set at 0.5%, which was broadly accepted throughout the industry.
These thresholds became obsolete when EDC enabled locations to submit data directly, eliminating the requirement for transcription. Nonetheless, it is the responsibility of data management teams to participate in several efforts to prepare data for acceptable analysis and submission.
The quality of the efforts that result in the development of data-collecting technologies and the scrubbing of collected data can have a direct influence on the quality of the data gathered. Thus, it is critical ...
... for organizations to consider managing the quality of the workstreams in which their teams participate, especially as we see increased streams of data being collected from various sources such as eSource, ePRO/eCOA, EMR/EHR, wearables, mHealth, and AI-based tools for adherence tracking, among others.
The old concept of an error rate is no longer an effective approach for managing quality expectations; rather, quality must be fostered as a habit or culture within data-handling teams. Teams must also use a qualitative approach to gauging quality rather than a quantitative effort of sample QA of the effort. The four treatment areas listed below should assist in building a quality culture:
1. Effective Review of Data Collection Tool (DCT) Design Specifications
Clinical trials are a form of "data collection." If we do not build the tool appropriately to gather data, we create a gap that cannot be filled, resulting in a pile-up of gaps with remedies, which results in teams putting in extra effort to assure data quality.
Specs are generally evaluated, but how efficiently are we looking at the suitability of the design from the standpoint of the site for EDC and the patient for ePRO? Patient-centricity is highly valued in the United States, because of regulations such as the 21st Century Cures Act, which improves data quality.
As a result, we should consider more patient-centric data-collecting requirements that can encourage sites and patients to submit accurate answers to the questions on respective Case Report Forms (CRFs). A patient with muscular dystrophy, for example, might be more interested in analyzing how well he or she can do daily tasks or play with their grandkids rather than measuring a 6-step walking test that must be reported on a regular basis.
2. Integrations
Eliminating manual interventions in data gathering is seen as the way of the future, with systems that enable EHR/EMR interfaces playing a key role. By integrating wearables and the mHealth tool, the use of medical-grade devices to capture data directly from patients would allow calibrated data to flow into integrated EDC databases with few or no interventions.
Without the need for human engagement, AI-powered technologies may collect drug adherence data. Moreover, integrating eCOAs, Central Lab APIs, Medical coding, Imaging, and safety data flows with EDCs would aid in centralized data collecting with little manual involvement in data transfer from various sources.
Utilizing EDC solutions in conjunction with supporting products such as eConsent, eCOA/ePRO, Imaging, Safety Gateway, and so on within the same architecture saves time and effort when setting up and monitoring integration. Overall, ensuring that the whole data flow requires minimum manual intervention might open up prospects for greater data quality.
3. Data Standardization
Automation of procedures for transforming obtained data to standards will improve both quality and efficiency. The approach begins with the development of CDISC-compliant eCRFs and ends with the implementation of standard mapping algorithms earlier in the project lifecycle than typical so that the SDTM needs during the study's execution are addressed smoothly and with increased quality.
This contributes to the streamlining of downstream statistical programming needs, making them more efficient, accurate, and consistent across many data releases within the same research or throughout a program or portfolio of studies.
4. Training & Knowledge Sharing
We all know that less human interaction leads to higher quality since it decreases the possibility of error; nevertheless, designing automation and integration to meet the goals established is vital. All systems must be set up such that everyone engaged has a better, broader, and deeper awareness of the end-to-end process flow.
General and study-level training are now merely part of the onboarding process. Gaining thorough awareness through excellent training is critical to ensuring that teams produce "first-time quality." Training should concentrate on features of good study design that are developed from a combination of technical and clinical knowledge.
An effective success measurement method for training and on-the-job mentoring programs might go a long way toward assuring data collecting quality. Companies should also support knowledge-sharing systems inside their infrastructure, allowing teams to build distinct learning communities.
In Summation
While adopting standard processes that comply with industry best practices is crucial to increasing clinical data collection and quality at your research organization, clinical trial efficiency is frequently only as good as the methods you choose to deploy.
When it comes to data management, electronic data capture (EDC) solutions should support rather than discourage corporate best practices for data quality. The finest EDC systems are simple to use and straightforward for all staff members, lowering the possibility of error while reporting into the system.
Your EDC system should be safe, reduce inappropriate data acquisition, and allow you to export your data properly. Certain systems, such as Octalsoft EDC, have features such as edit checks, visit and timepoint tolerances, and conditional forms, which help to ensure the accuracy of your clinical data.
Need an effective and efficient EDC system?
To reduce redundant data entry and error, Octalsoft EDC allows customers to create custom forms, set up edit checks, and use forms across several protocols. Discover how Octalsoft EDC may help you streamline your data collection, management, and compliance. Start now!
Add Comment
Health Articles
1. Which Is The Best Orthopedic Hospital In Jaipur?Author: Shreyaditya Hospital
2. Discover The Best Plastic Surgeons In The Chicago Area For Stunning, Safe Results
Author: Ciplasticsurgery
3. Choose Stallings Dental In St. Louis For Affordable Dental Implant Cost In Missouri
Author: Jessica Williams
4. The Best Hangover Cure And Essential Children's Vitamins For A Healthy Lifestyle
Author: michellumb44
5. Choosing The Right Altruistic Surrogacy Agency: Your Guide To Parenthood
Author: Surrogacy Centre India
6. Cyst Removal London - Cyst Removal Clinic London
Author: Revitalise London
7. Rhc Billing Audits: Proactive Steps For Financial Health
Author: Charlie Robinson
8. Individual Therapy Sessions: Benefits, Process | Snbcare
Author: Travis
9. Advantages And Disadvantages Of Fruits And Vegetables Wash
Author: Veg Fru Wash
10. Best Lasik Eye Surgery In Thane With Latest Technology
Author: Anil Eye Hospital
11. Do Adaptil Collars Work For Puppies?
Author: VetSupply
12. Get The Best Dental Implants In Camas Wa
Author: Camas Periodontics
13. How Long Does It Take For An Adaptil Calming Collar To Work?
Author: VetSupply
14. Neuro Rehabilitation Gurgaon: Expert Care For Faster Recovery
Author: neurolinkrehab
15. Best Centers For Autism Treatment In Hyderabad: Expert Guidance & Care
Author: neurolinkrehab