ALL >> Education >> View Article
Data Science With Generative Ai Course | Ameerpet
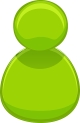
Data Science with Generative Ai: From Research to Real-World Applications
Data Science with Generative Ai Course has emerged as a transformative force in data science, blending advanced machine learning techniques with innovative applications. From enhancing research methodologies to solving real-world challenges, generative AI is revolutionizing industries. This article delves into its evolution, applications, and tips for effective use in data science.
Introduction to Generative AI in Data Science
Generative AI refers to a subset of artificial intelligence that can create new data based on existing datasets. Using algorithms like Generative Adversarial Networks (GANs) and Variational Autoencoders (VAEs), these models simulate realistic data, ranging from text and images to audio and videos.
In data science, generative AI has pushed boundaries by enhancing data processing, synthesis, and problem-solving. Whether for academic research or industry innovations, generative AI has enabled breakthroughs that were previously unattainable.
Key Components of Generative AI in Data Science
Generative ...
... AI’s integration into data science hinges on several foundational components:
• Data Generation: Generating synthetic datasets for model training and testing, especially when real-world data is scarce or sensitive.
• Predictive Analysis: Enabling models to predict future outcomes with better precision by simulating various scenarios.
• Data Augmentation: Improving the quality and quantity of datasets, leading to robust and versatile models.
• Algorithmic Innovation: Leveraging GANs, VAEs, and Transformer-based models like GPT for diverse applications.
Applications of Generative AI in Data Science
Generative AI is reshaping the data science landscape across industries.
Healthcare and Medical Research
• Synthetic Patient Data: Creating anonymized patient data for research while maintaining privacy.
• Drug Discovery: Generating molecular structures to speed up drug design and testing.
• Medical Imaging: Enhancing diagnostic tools by generating high-quality medical images.
Marketing and Customer Insights
• Content Personalization: Crafting personalized content for targeted advertising.
• Behavioral Predictions: Simulating customer behavior for market segmentation and forecasting.
• Visual Content Creation: Designing banners, logos, and product mockups using generative models.
Finance and Fraud Detection
• Risk Analysis: Simulating financial scenarios for investment strategies.
• Fraud Detection: Identifying fraudulent patterns by generating and analyzing anomalies in data. Data Science with Generative Ai Online Training
Entertainment and Media
• Content Creation: Generating scripts, music, and visual effects for movies and games.
• Virtual Reality (VR): Building immersive VR environments with realistic textures and objects.
• Image and Video Editing: Enhancing visual content using AI-driven tools.
Manufacturing and Design
• Product Prototyping: Creating design prototypes quickly and cost-effectively.
• Supply Chain Optimization: Generating simulation models for supply chain improvements.
Challenges in Using Generative AI in Data Science
Despite its potential, generative AI faces several challenges in practical applications:
• Data Bias: Models may inherit biases present in training datasets, leading to skewed results.
• Ethical Concerns: Issues like deepfake misuse and intellectual property theft raise ethical dilemmas.
• Computational Resources: Generative models often demand significant computational power.
• Accuracy and Validation: Ensuring the reliability and quality of generated data can be complex.
Future of Generative AI in Data Science
Generative AI is expected to grow exponentially in its influence on data science. Emerging trends include:
• Real-Time Applications: Advancements in real-time generative systems for adaptive learning.
• Explainable AI: Developing tools to make generative models more transparent and interpretable.
• Cross-Disciplinary Innovations: Applying generative AI in interdisciplinary fields like bioinformatics and environmental science.
Tips for Implementing Generative AI in Data Science
To effectively leverage generative AI, data scientists should adopt the following practices:
Start with Clear Objectives
• Define the goals for implementing generative AI.
• Focus on areas where traditional methods fall short, such as data scarcity or model enhancement.
Use Quality Datasets
• Ensure datasets are diverse, unbiased, and representative of the intended application.
• Regularly audit and clean data to avoid model errors.
Select the Right Algorithms
• Choose algorithms tailored to the task, such as GANs for image generation or VAEs for dimensionality reduction.
• Stay updated on emerging models for improved efficiency and accuracy.
Monitor and Validate Outputs
• Continuously validate generated data for reliability and relevance.
• Use domain-specific benchmarks to assess model performance.
Prioritize Ethical Considerations
• Implement safeguards against misuse, such as watermarking generated content.
• Comply with data privacy regulations and ethical AI standards.
Invest in Training and Collaboration
• Upskill teams with specialized training in generative AI tools. Data Science with Generative Ai Training
• Collaborate with cross-functional experts to enhance the scope of applications.
Conclusion
Generative AI is a pivotal force in data science, enabling innovation across diverse domains. From synthesizing data for research to solving industry challenges, its applications are vast and transformative. However, with great power comes responsibility. Adopting best practices, ensuring ethical use, and addressing challenges head-on are essential for harnessing its full potential.
Visualpath Advance your career with Data Science with Generative Ai Course. Gain hands-on training, real-world skills, and certification. Enroll today for the best Data Science with Generative Ai. We provide to individuals globally in the USA, UK, etc.
Call on: +91 9989971070
Course Covered:
Data Science, Programming Skills, Statistics and Mathematics, Data Analysis, Data Visualization, Machine Learning,
WhatsApp: https://www.whatsapp.com/catalog/919989971070/
Blog link: https://visualpathblogs.com/
Visit us: https://www.visualpath.in/online-data-science-with-generative-ai-course.html
Add Comment
Education Articles
1. Full Stack Course In Digital MarketingAuthor: education
2. Master Digital Marketing With Talentkaksha
Author: Digital Marketing with TalentKaksha
3. Top 10 Usa Study Abroad Consultants - Disha Consultants
Author: anas saifi
4. Master Digital Marketing With Talentkaksha Online Course
Author: TalentKaksha Online Course
5. How To Choose The Right Study Abroad Courses For Indian Students
Author: Videsh
6. Ayurveda Therapy Course - Asap Kerala
Author: rithika
7. Azure Ai-102 Course In Hyderabad | Azureai Engineer Training
Author: gollakalyan
8. Oracle Cloud Infrastructure Training And Certification
Author: SIVA
9. Genai Training | Generative Ai Online Training Courses
Author: Anika Sharma
10. Visual Storytelling: Using Graphics To Drive Digital Marketing Success
Author: Rajat Sancheti
11. Sre Online Training | Site Reliability Engineering Training
Author: krishna
12. Qqi Level 5 Healthcare Support Major Award: A Pathway To A Rewarding Career In Healthcare
Author: johnnytorrt
13. Everything You Need To Know About Hse Professional Training
Author: Emma
14. Salesforce Devops Training | Salesforce Devops Online Training
Author: himaram
15. Achieve Balance Between Style And Functionality With Interior Design Education And Practical Skills
Author: raseenadm