ALL >> Technology,-Gadget-and-Science >> View Article
Due Diligence Report: Common Challenges In Ai Assessments
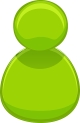
Creating an effective due diligence report for AI assessments is essential but complex, requiring awareness of several challenges unique to AI technologies. Below are some of the most common challenges that teams encounter ...
Challenge 1: Assessing Data Quality and Relevance
Data quality is central to the functionality and trustworthiness of AI models, but it’s often difficult to evaluate comprehensively. Poor data can lead to biased or unreliable outcomes, yet not all datasets used in training are transparent or traceable. During the creation of a report after due diligence, assessing the quality and relevance of the data used in AI models is essential.
Challenge 2: Evaluating Algorithm Transparency
One of the toughest challenges in AI due diligence is ensuring algorithm transparency, particularly with complex models like deep neural networks. These models often function as “black boxes,” making it hard to interpret the logic behind their predictions. A report following due diligence should examine whether the AI model has built-in explainability ...
... features, as these are crucial for sectors requiring high accountability, like healthcare or finance.
Challenge 3: Estimating Long-Term Maintenance and Scalability
Predicting long-term maintenance needs is tricky, yet essential, for accurate AI assessments. AI models frequently require updates as data changes or as business needs evolve. Evaluating scalability is also important; without testing, it’s hard to foresee if an AI model can handle larger datasets or increased user demand. A due diligence report should outline the resources and infrastructure needed to maintain the AI’s functionality over time.
Challenge 4: Navigating Intellectual Property (IP) Concerns
With AI, intellectual property rights can become complicated, especially if the model uses proprietary data or was built on open-source algorithms with restrictive licenses. Overlooking IP concerns can lead to future legal or financial risks. A comprehensive report developed after due diligence should identify any potential IP issues, ensuring there’s clarity on data ownership and AI model licensing.
Challenge 5: Addressing Regulatory Compliance
AI systems are subject to regulations such as GDPR for data privacy in Europe or HIPAA in healthcare in the U.S. Compliance is especially challenging for international AI systems operating across multiple jurisdictions with different laws. It’s crucial to review the AI system’s data handling, storage, and processing protocols to ensure they meet the necessary compliance standards.
Challenge 6: Identifying Security Vulnerabilities
AI models are vulnerable to security risks, such as data breaches or adversarial attacks that manipulate input data to trick the model. Security gaps can compromise not only the AI’s integrity but also the organization’s reputation. During due diligence, a thorough assessment of the AI’s security measures is necessary to flag exploitation vulnerabilities.
Challenge 7: Mitigating Model Bias
Bias in AI is a persistent issue, as AI models often mirror the biases present in their training data. This can lead to unfair or discriminatory outcomes, which could harm both users and brand reputation. The due diligence report should evaluate the AI system’s approach to bias mitigation, including any methods it employs to detect and reduce bias across different user demographics.
Challenge 8: Evaluating Integration with Existing Systems
AI tools don’t always integrate smoothly with existing or legacy business systems with outdated infrastructure. Poor integration can create operational challenges and impact user experience. Assessing integration during due diligence can help avoid unforeseen costs and delays, ensuring that the AI system can seamlessly support and enhance the current technological environment.
Challenge 9: Measuring Return on Investment (ROI)
Calculating the ROI of an AI model can be challenging, as returns are not always immediate and may be hard to quantify … for instance, improvements in efficiency or customer experience. A due diligence report should consider potential qualitative and quantitative benefits, providing stakeholders with a balanced view of the AI system’s long-term value.
Add Comment
Technology, Gadget and Science Articles
1. How Online Production Planning Shapes The Manufacturing Realm?Author: logitrac360
2. Web Scraping Ecommerce Data For Retail Price Comparison In 2024
Author: Devil Brown
3. 2025 Web Design Trends In Vietnam - Perfectiongeeks Expertise
Author: Perfectiongeeks
4. The Role Of Translation Services In Global Business Expansion
Author: premiumlinguisticservices
5. Admission Crm Software For Colleges And Universities
Author: Aditya Sharma
6. Unlock The Full Potential Of Your Komatsu Equipment With Comprehensive Manuals
Author: Heavy Equipment Manual
7. The Role Of Training Tracker Software In Ensuring Workplace Compliance
Author: Siva Harish K P
8. Effortless Resource Planning With Odoo: Drive Productivity Through Workforce Optimisation
Author: Alex Forsyth
9. How New Trends In Pharma Marketing Are Shaping The Future
Author: Jesvira
10. What Is A Transparent Led Display And How Does It Revolutionize Outdoor Advertising?
Author: ledcontrollercard
11. Here’s How Smart Locks Provide One Less Thing To Worry About
Author: Nigam
12. The Impact Of Digital Transformation On Retail Innovation Efficiency
Author: Sparity
13. Cloud Computing Trends To Watch Out In 2025
Author: Sparity
14. Mastering Web Development Certifications
Author: EDCHART
15. Nurturing Joy: The Advantages Of Managing Your Vegetable Garden Effectively
Author: James