ALL >> Education >> View Article
The Top 10 Challenges In Data Science Today
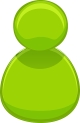
Data science has revolutionized the way organizations operate, unlocking valuable insights from vast amounts of data. However, as data science evolves, professionals in this field face a range of challenges. These challenges come from various aspects, including technical complexities, ethical concerns, and the sheer scale of data. Let's explore the top 10 challenges in data science today.
1. Data Quality and Availability
One of the most fundamental challenges in data science is ensuring data quality and availability. Bad data—whether incomplete, inconsistent, or inaccurate—can significantly skew the results of data analysis. Often, data is missing or collected incorrectly, making it unreliable for modeling.
To combat this, data scientists spend a significant portion of their time cleaning and preparing data. This process, known as data wrangling, involves filling in missing values, correcting errors, and normalizing the data format. Without high-quality data, even the most sophisticated algorithms won’t deliver valuable insights.
2. Handling Big Data
With the exponential growth of data ...
... from multiple sources, handling big data has become a daunting task. Big data is characterized by its high volume, velocity, and variety, making traditional data processing techniques insufficient.
Data scientists must use advanced tools and platforms like Hadoop, Apache Spark, and distributed cloud-based storage solutions to handle the massive datasets generated by businesses today. However, the cost and complexity of managing this infrastructure can be overwhelming, particularly for smaller organizations.
3. Integration of Data from Multiple Sources
Another major challenge is integrating data from various sources. Organizations often collect data from different systems, including social media platforms, IoT devices, internal databases, and external APIs. The challenge arises when trying to merge data in different formats, structures, and levels of completeness into a unified system for analysis.
Effective data integration requires robust Extract, Transform, Load (ETL) processes, which standardize data before it can be analyzed. However, this can be time-consuming, and improper integration may result in data loss or inaccurate insights.
4. Privacy and Security Concerns
With the surge in data collection comes the challenge of maintaining privacy and security. Data scientists often deal with sensitive information like personal identifiable information (PII), financial details, and health records. This data must be safeguarded to avoid breaches, which could lead to legal penalties and loss of trust from customers.
Laws like the General Data Protection Regulation (GDPR) and the California Consumer Privacy Act (CCPA) set stringent rules regarding the use and storage of personal data. Navigating these regulations while trying to perform complex data analyses is a critical challenge in the field.
5. Interpretability of Results
Data science is not just about generating insights; it's also about communicating those insights effectively to non-technical stakeholders. Many advanced models, like deep learning algorithms, are often seen as "black boxes" because their internal workings are difficult to interpret.
For businesses, it’s crucial to understand why a model made a certain prediction or recommendation. If the results are not interpretable, decision-makers may hesitate to trust the model's outcomes. The challenge here is balancing the complexity of models with their interpretability.
6. Bias and Fairness in Algorithms
Algorithmic bias is a significant challenge in data science. Models can unintentionally perpetuate societal biases if the data used to train them is skewed or incomplete. For example, an algorithm designed to screen job applications might favor candidates from certain demographics if the training data itself is biased toward those groups.
Ensuring fairness in algorithms is critical, especially in industries like finance, healthcare, and recruitment. Data scientists need to develop techniques to identify and eliminate bias in their models, ensuring that predictions are equitable for all groups.
7. Lack of Skilled Professionals
There is a high demand for data scientists, but the supply of skilled professionals has not kept pace. The complexity of data science requires expertise in mathematics, statistics, programming, and domain-specific knowledge. Finding individuals who possess all these skills is a challenge for organizations.
Moreover, the rapid evolution of tools and technologies means that data scientists must continuously learn and adapt. Companies are investing in training programs to upskill their current workforce, but this can be a slow and costly process.
8. Model Deployment and Scalability
Once a model has been developed and tested, deploying it into a real-world environment presents another set of challenges. Scaling a model to handle large volumes of real-time data, integrating it with existing systems, and ensuring that it performs well under different conditions are not straightforward tasks.
Model deployment often requires collaboration between data scientists, software engineers, and IT teams. Even after deployment, monitoring the model's performance and updating it regularly to account for new data is essential. This entire process can be time-consuming and resource-intensive.
9. Keeping Up with Rapid Technological Changes
The field of data science is evolving at an unprecedented rate. New tools, algorithms, and platforms are being developed constantly. While this opens up new possibilities, it also poses a challenge for data scientists, who must continuously stay updated with the latest developments.
For instance, advancements in machine learning frameworks like TensorFlow and PyTorch, as well as the emergence of AutoML (automated machine learning) tools, have simplified some aspects of data science. However, mastering these tools requires continuous learning and adaptation, which can be overwhelming, especially for professionals balancing multiple responsibilities.
10. Ethical Dilemmas in Data Usage
Ethics is a growing concern in data science. While data has the power to drive positive change, it can also be misused in ways that harm individuals or groups. For example, predictive algorithms can sometimes reinforce harmful stereotypes, or data can be used for invasive surveillance.
Data scientists must navigate these ethical dilemmas, ensuring that their work aligns with principles of fairness, transparency, and responsibility. Ethical considerations should be part of the entire data science workflow—from data collection to model deployment.
Many organizations are now establishing ethical guidelines and review boards to oversee data science projects. However, these processes are still in their infancy, and balancing ethical concerns with business objectives remains a significant challenge.
Conclusion
Data science continues to be a transformative field, offering incredible opportunities for innovation across industries. However, it is not without its challenges. From data quality issues to ethical concerns, data scientists must navigate a complex landscape to extract meaningful insights and build reliable models. For those aspiring to join this dynamic field, pursuing a Data Science course in Lucknow
, Nagpur, Delhi, Noida, and more cities in India provides a solid foundation for mastering the essential skills and technologies.
Addressing these challenges requires collaboration between data scientists, engineers, business leaders, and policymakers. As technology continues to advance, overcoming these hurdles will become even more critical for ensuring that data science delivers on its promise responsibly and effectively.
Add Comment
Education Articles
1. Gavin Mccormack Journey As An Education ChangemakerAuthor: selinclub
2. What Makes Dubai An Ideal Destination For Global Business Conferences?
Author: All Conference Alert
3. D365 Functional Course In Ameerpet | Dynamics 365 Course
Author: Hari
4. Best Sre Certification Course | Sre Training Online In Bangalore
Author: krishna
5. Best Google Cloud Ai Training In Ameerpet | Visualpath
Author: visualpath
6. Azure Ai Engineer Course In Bangalore | Azure Ai Engineer
Author: gollakalyan
7. What To Expect At The Vermont Dmv Driving Test
Author: Ravinder Malik
8. Key Highlights Of Punyam Academy’s Iso 9001 Lead Auditor Training Course
Author: Emma
9. Ai With Aws Training | Ai With Aws Online Training Bangalore
Author: naveen
10. Salesforce Devops Training | Salesforce Devops With Copado
Author: himaram
11. How Does Cpr Affect High-risk Professions Like Healthcare, Sports, And More?
Author: Christopher Bayer
12. Best Bba Colleges In Hyderabad For Students Seeking A Corporate Career
Author: SSDC
13. Why We Charge A Training Fee At Pydun Technology
Author: Pydun Technology Private Limited
14. Informatica Idmc | Informatica Online Training In Hyderabad
Author: gollakalyan
15. Best Snowflake Course | Snowflake Training In India
Author: Pravin