ALL >> Business >> View Article
The Importance Of Data Sourcing In Machine Learning
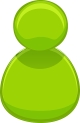
Introduction to Machine Learning
Machine learning is revolutionizing the way we approach problems and make decisions. From self-driving cars to personalized recommendations on streaming services, it’s changing our lives in remarkable ways. But at the heart of every successful machine learning model lies one crucial element: data. Without quality data, even the most advanced algorithms can fall flat.
This brings us to data sourcing—a vital process that shapes the effectiveness of machine learning initiatives. In an age where information is abundant, understanding how to source relevant and high-quality data sets becomes imperative for any organization looking to harness the power of machine learning. Let’s dive deeper into this essential aspect and explore its significance in creating robust models that deliver real value.
What is Data Sourcing and Why is it Important?
Data sourcing refers to the process of gathering and selecting data necessary for building machine learning models. It involves identifying relevant datasets that can provide the insights needed to train algorithms effectively. ...
...
The importance of data sourcing cannot be overstated. Quality data is the foundation upon which successful machine learning projects are built. Without it, even the most sophisticated algorithms will struggle to perform accurately.
Data quality directly impacts model performance. Accurate and diverse datasets lead to better predictions and more reliable outcomes in various applications, from healthcare to finance.
Additionally, proper data sourcing services ensures compliance with privacy regulations. This aspect is crucial as it safeguards user information while still providing valuable insights for analysis.
In a rapidly evolving technological landscape, effective data sourcing helps organizations stay competitive by leveraging their unique datasets for innovative solutions.
Challenges of Data Sourcing in Machine Learning
Data sourcing in machine learning is fraught with challenges that can undermine project success. One major hurdle is data quality. Inaccurate or inconsistent data can lead to misleading outcomes and faulty predictions.
Another challenge involves access to relevant datasets. Many organizations grapple with finding the right type of data that aligns with their specific objectives, leading to potential gaps in information.
Additionally, compliance issues are prevalent. Regulations like GDPR impose strict guidelines on how personal data should be handled, creating barriers for many companies trying to source useful information without risking legal implications.
The sheer volume of available data can be overwhelming. Sifting through vast amounts of unstructured or semi-structured content to identify valuable insights requires significant time and resources, often stretching teams thin.
Types of Data Sources for Machine Learning
When it comes to data sourcing for machine learning, diversity is key. Different types of data sources can significantly impact the performance of your models.
Structured data is one common type. This includes organized datasets like spreadsheets and databases, where information is easily accessible and analyzable.
Unstructured data presents a different challenge. Think about text documents, images, or videos that require more processing to extract valuable insights.
Another source is semi-structured data, which combines elements from both structured and unstructured formats. JSON files or XML documents fall into this category, offering flexibility in how they can be analyzed.
Public datasets are also worth exploring. Many organizations share their findings online—great opportunities for acquiring quality data without high costs.
Synthetic data has emerged as an innovative option. Created through algorithms rather than collected from real-world scenarios, it allows researchers to simulate various conditions effectively while protecting privacy concerns.
Best Practices for Data Sourcing in Machine Learning
When it comes to data sourcing, quality is paramount. Start by clearly defining your objectives. Understand what you need the data for and how it will impact your machine learning model.
Next, prioritize diversity in your datasets. A variety of sources can help avoid bias, making models more robust and reliable. Don't hesitate to mix structured and unstructured data for a comprehensive approach.
Documentation is another critical aspect often overlooked. Keep track of where each dataset originates from, including any transformations applied during processing. This promotes transparency and facilitates easier troubleshooting later on.
Regularly revisit your sourced data as well. Data can change over time; continuous evaluation ensures that you're using the most relevant information available.
Collaboration with domain experts enhances the relevance of the data sourced. Their insights can guide you toward valuable datasets that may not be immediately obvious but are essential for effective modeling.
Real-World Examples of Successful Data Sourcing in Machine Learning
Companies across various industries illustrate the power of effective data sourcing in machine learning.
Consider Netflix. They leverage viewer data to refine their recommendation algorithms. By analyzing user preferences, they enhance content delivery, ensuring subscribers receive personalized suggestions that keep them engaged.
In healthcare, IBM Watson Health demonstrates another success story. The platform sources vast medical datasets to aid in diagnostics and treatment recommendations. This rich pool of information enhances decision-making for doctors and improves patient outcomes.
Retail giants like Amazon also excel at data sourcing. Their use of customer behavior analytics helps optimize inventory management and target marketing campaigns effectively. This strategy drives sales while catering precisely to consumer needs.
These examples highlight how strategic data sourcing can transform operations across sectors, leading to innovative solutions and improved service delivery.
Conclusion: The Impact of Quality Data on Machine Learning Models
Quality data plays a pivotal role in the effectiveness of machine learning models. When sourced correctly, it forms the backbone of any successful project. Models trained on high-quality datasets are more accurate and reliable.
The nuances of data sourcing can significantly influence outcomes. A well-sourced dataset enriches the model's ability to learn patterns and provide valuable insights. On the other hand, poor data quality leads to skewed results and unreliable predictions.
Understanding that not all data is created equal is essential for practitioners in this field. Prioritizing quality over quantity during the sourcing process can save time, resources, and effort down the line.
As businesses increasingly rely on machine learning technologies for decision-making, investing in robust data sourcing strategies becomes paramount. The impact of these strategies can be seen across various industries where innovative solutions hinge on effective machine learning applications fueled by exceptional datasets. Quality matters—it's what drives success in this rapidly evolving landscape.
Add Comment
Business Articles
1. Lucintel Forecasts The Glass Fiber In The Global Marine Market To Grow With A Cagr Of 3.7% From 2024 To 2030Author: Lucintel LLC
2. Lucintel Forecasts The Glass Fiber In The Global E&e Market To Grow With A Cagr Of 3.9% From 2024 To 2030
Author: Lucintel LLC
3. Lucintel Forecasts The Global Frp Pole In Telecommunication Market To Grow With A Cagr Of 6.5% From 2024 To 2030
Author: Lucintel LLC
4. Lucintel Forecasts The Global Frp Pole In Power Transmission & Distribution Market To Grow With A Cagr Of 6% From 2024 To 2030
Author: Lucintel LLC
5. Lucintel Forecasts The Global Frp Pipe In Oil And Gas Market To Grow With A Cagr Of 5.1% From 2024 To 2030
Author: Lucintel LLC
6. Lucintel Forecasts The Global Frp Pipe In Chemical Market To Grow With A Cagr Of 3.6% From 2024 To 2030
Author: Lucintel LLC
7. Adopt A Modern Telecom Commission Management System To Fix Revenue Leakages
Author: Kevin
8. Quality Steel Pipes And Tubes With Commitment And Trusted Service
Author: CONTENT EDITOR FOR SAMPHIRE IT SOLUTIONS PVT LTD
9. Lucintel Forecasts The Global Foam Core For The Construction Market To Grow With A Cagr Of 3.7% From 2024 To 2030
Author: Lucintel LLC
10. How Important Is Mutual Fund?
Author: Sagar Shah
11. What Do You Need To Know About Customize Your Own Clothes
Author: Guangzhou Beianji Clothing
12. Detailed Tips To Choose Quality Cabinets And Joinery Melbourne
Author: William Harvey
13. Lucintel Forecasts The Global Foam Core For The Transportation Market To Grow With A Cagr Of 5.4% From 2024 To 2030
Author: Lucintel LLC
14. Silicone Sealing Foam Cord: The Valuable Option For You
Author: Dongguan Senma New Materials Technology Co., Ltd
15. How Investment Banking Companies And Credit Solutions Elevate Wealth Management
Author: Drishti Desai