ALL >> Education >> View Article
Data Science In Fraud Analytics
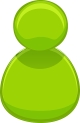
Fraud is a pervasive issue that affects various sectors, including finance, retail, and insurance. The ability to detect and prevent fraudulent activities is crucial for safeguarding assets and maintaining trust. Data science plays a pivotal role in modern fraud analytics, offering sophisticated tools and techniques to identify, analyze, and mitigate fraudulent behaviors.
Understanding Fraud Analytics
Fraud analytics involves using data analysis techniques to identify suspicious activities and prevent fraud. This process relies heavily on data science, which provides the methodologies and tools needed to detect anomalies, predict potential fraud, and enhance overall security.
The Role of Data Science in Fraud Detection
Data science is at the core of effective fraud detection. By leveraging large datasets and advanced algorithms, data science helps in uncovering patterns and anomalies that indicate fraudulent activities. Machine learning models and statistical techniques are employed to analyze transaction data, user behaviors, and historical fraud patterns.
Machine Learning for Fraud Detection
...
... Machine learning algorithms are crucial for detecting fraud in real-time. These algorithms analyze historical data to build models that can predict fraudulent activities. Techniques such as supervised learning, unsupervised learning, and anomaly detection are used to identify unusual patterns and flag potentially fraudulent transactions.
Enrolling in a data science institute can provide the foundational knowledge needed to understand and implement these machine learning techniques effectively. Such courses cover essential topics like feature engineering, model training, and evaluation, which are critical for developing robust fraud detection systems.
Predictive Analytics and Anomaly Detection
Predictive analytics involves using historical data to forecast future fraudulent activities. By analyzing patterns from past fraud incidents, data scientists can build models that predict the likelihood of fraud occurring in new transactions. Anomaly detection algorithms further enhance this process by identifying deviations from expected behavior, which may indicate fraudulent activities.
A data scientist course offers insights into predictive modeling and anomaly detection, equipping individuals with the skills to create and refine models that improve fraud detection capabilities.
Enhancing Fraud Prevention Strategies
Effective fraud prevention requires a proactive approach, combining data science techniques with strategic planning. By analyzing data from various sources, organizations can develop strategies to minimize the risk of fraud and respond swiftly to suspicious activities.
Risk Assessment and Scoring
Data science facilitates risk assessment by analyzing data to identify high-risk entities and transactions. Risk scoring models assign scores to transactions based on their likelihood of being fraudulent. These models use historical data, user behavior, and contextual information to evaluate risk levels.
Learning to build and implement risk scoring models is a key component of a data science course. Such courses cover various techniques for creating effective scoring systems and integrating them into fraud prevention strategies.
Real-Time Fraud Detection Systems
Real-time fraud detection systems are essential for immediate response to suspicious activities. Data science enables the development of systems that analyze transaction data in real time, flagging potentially fraudulent activities as they occur. These systems rely on real-time data feeds and advanced algorithms to ensure timely detection and prevention.
A data science course can provide the skills needed to design and implement real-time fraud detection systems, including knowledge of data streaming, real-time analytics, and system integration.
Case Studies in Fraud Analytics
Examining real-world applications of data science in fraud analytics helps illustrate its impact and effectiveness. Here are some examples of how data science is used to combat fraud:
Case Study 1: Credit Card Fraud Detection
Credit card companies use data science to detect fraudulent transactions by analyzing spending patterns, transaction frequencies, and user behaviors. Machine learning models help identify unusual transactions that deviate from a user’s typical behavior, allowing for quick intervention and fraud prevention.
Case Study 2: Insurance Fraud Detection
Insurance companies employ data science to detect fraudulent claims by analyzing historical claim data and identifying patterns associated with fraudulent activities. Predictive analytics and anomaly detection are used to flag suspicious claims and prevent fraudulent payouts.
Case Study 3: E-Commerce Fraud Prevention
E-commerce platforms use data science to combat fraudulent activities such as account takeovers and fraudulent purchases. By analyzing transaction data, user behavior, and device information, these platforms can identify and prevent fraudulent transactions in real time.
Future Trends in Fraud Analytics
The field of fraud analytics is continuously evolving, driven by advancements in data science and technology. Here are some emerging trends to watch:
Integration of Artificial Intelligence (AI)
AI is increasingly being integrated into fraud analytics to enhance detection capabilities. AI-powered systems can analyze complex datasets and identify subtle patterns that may indicate fraud. Natural language processing and computer vision are also being used to analyze unstructured data and detect fraud.
Use of Blockchain Technology
Blockchain technology offers a decentralized and secure approach to data management. By leveraging blockchain for transaction verification and auditing, organizations can enhance transparency and reduce the risk of fraud.
Advances in Data Privacy and Security
As data privacy regulations become more stringent, fraud analytics will need to adapt to ensure compliance while maintaining effective detection capabilities. Data science will play a crucial role in developing secure and privacy-conscious fraud detection systems.
Data science is transforming fraud analytics by providing advanced tools and techniques to detect and prevent fraudulent activities. From machine learning and predictive analytics to real-time fraud detection systems, data science enables organizations to safeguard their assets and maintain trust. Enrolling in a data science course can equip individuals with the skills needed to excel in this field, offering insights into the methodologies and technologies that drive effective fraud detection. As fraud tactics continue to evolve, data science will remain a vital component in combating fraud and ensuring security.
Add Comment
Education Articles
1. Can Ielts Academic Be Used For Immigration?Author: lily bloom
2. A Course On The Importance Of Upskilling At The College Level
Author: stem-xpert
3. Global Clinical Trials: Unveiling India’s Booming Market Outlook For 2025!
Author: Aakash jha
4. The Power Of Micro-influencers: Why They Matter More Than Ever
Author: dev
5. Digital Marketing Course In Dadar
Author: Sanchi
6. The Best Sre Course Online In India | Sre Training
Author: krishna
7. Top Google Cloud Ai Training In Chennai - Visualpath
Author: visualpath
8. Best Dynamics 365 Business Central Online Training - Hyderabad
Author: Susheel
9. The Best Data Engineering Course In Hyderabad - 2025
Author: naveen
10. Microsoft Dynamics 365 Course In Hyderabad | D365 Training
Author: Hari
11. Top Salesforce Marketing Cloud Training In Ameerpet
Author: Visualpath
12. Top Snowflake Course In Ameerpet | Snowflake Online Training
Author: Pravin
13. List Of Top Online Ma Colleges In India
Author: Study Jagat
14. Scrum Master Training In Bangalore | Scrum Master Classes
Author: visualpath
15. Mendix Online Training | Mendix Online Certification Course
Author: himaram