ALL >> Education >> View Article
Feature Engineering: Enhancing Data Analytics Insights
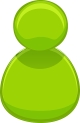
In the realm of data science, where insights drive decisions and innovations, feature engineering stands as a cornerstone of analytical prowess. It is not merely a technical process but rather an art form that transforms raw data into meaningful predictors, enriching the predictive power of machine learning models. This article delves into the significance of feature engineering in data analytics, its methodologies, and its pivotal role in shaping robust analytical outcomes.
Understanding Feature Engineering
At its essence, feature engineering involves the creation and selection of relevant features from raw data to enhance the performance of machine learning algorithms. These features serve as the input variables that algorithms utilize to make predictions or classifications. While algorithms play a crucial role in data analysis, their effectiveness hinges greatly on the quality and relevance of the features provided to them. Thus, feature engineering bridges the gap between raw data and actionable insights.
The Art and Science Behind Feature Engineering
Effective feature engineering requires a blend ...
... of domain knowledge, creativity, and technical expertise. It starts with a deep understanding of the data and the problem at hand. For instance, in a predictive maintenance scenario for industrial machinery, relevant features could include historical maintenance records, environmental factors, and operational metrics. Identifying and extracting such features necessitates collaboration between data scientists and domain experts to ensure that the engineered features are not only predictive but also interpretable and actionable.
Methodologies in Feature Engineering
Several methodologies exist to engineer features depending on the nature of the data and the objectives of the analysis. One common approach is transformation, where raw data is manipulated to create new features that better represent patterns in the data. For example, transforming a continuous variable into categories based on predefined thresholds can reveal non-linear relationships that are crucial for certain types of predictions.
Another powerful technique is feature scaling, which standardizes numerical features to a common scale, preventing algorithms from being biased towards features with larger numerical ranges. This is particularly important in algorithms like k-nearest neighbors or support vector machines where distance metrics play a significant role.
Moreover, dimensionality reduction techniques such as principal component analysis (PCA) or feature selection algorithms like recursive feature elimination (RFE) help streamline models by focusing on the most informative features while discarding redundant or irrelevant ones. These techniques not only improve model performance but also enhance computational efficiency, making them indispensable in large-scale data analytics.
Impact of Feature Engineering on Model Performance
The impact of feature engineering on model performance cannot be overstated. By providing models with more relevant and discriminative features, engineers can significantly enhance prediction accuracy, reduce overfitting, and improve generalization to unseen data. In the competitive landscape of data analytics, where marginal gains in accuracy can translate into substantial business advantages, effective feature engineering becomes a critical differentiator.
Challenges and Considerations
Despite its transformative potential, feature engineering is not without its challenges. One common pitfall is overfitting, where engineered features capture noise or irrelevant patterns from the training data, leading to poor performance on new data. To mitigate this risk, techniques such as cross-validation and regularization are employed to ensure that engineered features generalize well to unseen data.
Moreover, the iterative nature of feature engineering demands continuous evaluation and refinement. As data evolves and new insights emerge, engineers must adapt their feature selection strategies to maintain the relevance and predictive power of their models. This iterative process underscores the dynamic nature of data analytics and the continuous quest for optimal solutions.
Future Directions and Innovations
Looking ahead, advancements in artificial intelligence and machine learning promise to reshape the landscape of feature engineering. Techniques such as automated feature generation and selection, powered by deep learning algorithms, are poised to streamline the feature engineering process further. These innovations hold the potential to democratize data analytics certification, empowering organizations of all sizes to derive actionable insights from their data with unprecedented efficiency and accuracy.
Feature engineering stands as a linchpin of effective data analytics, bridging the gap between raw data and actionable insights. By transforming raw data into meaningful predictors, feature engineers empower machine learning models to make informed predictions and classifications. As data continues to proliferate and businesses increasingly rely on data-driven decision-making, the role of feature engineering will only grow in importance. It is not merely a technical endeavor but a strategic imperative for organizations seeking to harness the full potential of their data. As such, mastering the art and science of feature engineering is essential for aspiring data analysts and professionals in the field of data science.
As you embark on your journey into the realm of data analytics, whether through data analytics courses or self-study, remember that proficiency in feature engineering can unlock new dimensions of insight and innovation. Embrace the challenges, hone your skills, and explore the vast possibilities that feature engineering offers in transforming data into actionable intelligence.
My name is Gour and I am a technical content writer for DataMites. DataMites provides Artificial Intelligence, Data science, Machine learning and Python Programming Courses.
Add Comment
Education Articles
1. Devops: The Modern Skillset Every Tech Professional Should MasterAuthor: safarisprz01
2. Salesforce Marketing Cloud Training In India | Cloud
Author: Visualpath
3. How An English Medium School Shapes A Child’s Future In Today’s Global World
Author: Mount Litera Zee School
4. Mern Stack Online Training In Ameerpet | Mern Stack Ai Training
Author: Hari
5. Why Online Courses In Sap Sd Are The Best Solution For Today's Professionals
Author: ezylern
6. Sailpoint Online Course In Bangalore For Professionals
Author: Pravin
7. Sap Ai Course | Sap Ai Online Training In Hyderabad
Author: gollakalyan
8. Why Aima Is The Best Choice For A Global Advanced Management Programme
Author: Aima Courses
9. The Best Oracle Integration Cloud Online Training
Author: naveen
10. Mlops Training Course In Chennai | Mlops Training
Author: visualpath
11. International Cbse School In Nallagandla,
Author: Johnwick
12. Best Mba Dual Specialization Combinations For 2025 And Beyond
Author: IIBMS Institute
13. Top Docker Kubernetes Training In Hyderabad | Docker And Kubernetestop Docker Kubernetes Training In Hyderabad | Docker And Kubernetes
Author: krishna
14. Full Stack Web Development Course In Noida
Author: Training Basket
15. Master Advanced Pega Skills With Pega Cssa Infinity'24.2 Online Training By Pegagang
Author: PegaGang