ALL >> Education >> View Article
Bayesian Methods
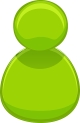
In the realm of data science, where the need to derive meaningful insights from vast amounts of data is paramount, Bayesian methods offer a powerful framework. Unlike traditional statistical approaches, which often rely on fixed parameters and assumptions, Bayesian statistics provides a flexible and intuitive way to update beliefs and make predictions based on new evidence. This blog post delves into the fundamentals of Bayesian methods, their applications in data science, and why they are increasingly becoming a valuable skill in today's data-driven world.
Understanding Bayesian Methods
Bayesian methods are rooted in the concept of Bayesian inference, named after the 18th-century mathematician Thomas Bayes. At its core, Bayesian inference allows us to update our beliefs about uncertain quantities as new data becomes available. This iterative process is particularly useful in data science for making predictions and decisions in the face of uncertainty.
Bayesian Inference Process
The process begins with prior beliefs, represented by a prior probability distribution, which encapsulates what is known about ...
... a quantity before observing any data. As new data is collected, these prior beliefs are updated using Bayes' theorem to form a posterior distribution, which combines the prior beliefs with the likelihood of the observed data given the parameters of the model. This posterior distribution then becomes the updated belief about the quantity of interest, incorporating both prior knowledge and new evidence.
Applications in Data Science
In the context of data science, Bayesian methods find application across various domains:
Predictive Modeling: Bayesian regression and classification techniques are widely used for predictive modeling tasks. These methods allow for the incorporation of prior knowledge about the relationships between variables, which can improve the accuracy and robustness of predictions.
Parameter Estimation: Bayesian inference provides a coherent framework for estimating model parameters, such as means, variances, or coefficients, by integrating prior beliefs with observed data. This approach is particularly useful when dealing with small datasets or when prior information about parameters is available.
Decision Making: Bayesian decision theory helps data scientists make optimal decisions under uncertainty by considering the costs and benefits associated with different actions. This framework is valuable in fields like healthcare, finance, and marketing, where decisions must be made based on incomplete or noisy data.
Anomaly Detection: Bayesian methods can be effective in identifying anomalies or outliers in data by modeling normal behavior and detecting deviations from the expected patterns. This capability is crucial for fraud detection, cybersecurity, and quality control in manufacturing.
Time Series Analysis: Bayesian techniques offer powerful tools for analyzing time series data, such as forecasting future values, identifying trends, and quantifying uncertainties. These methods are essential in fields like finance, climate science, and epidemiology, where understanding patterns over time is critical.
Advantages of Bayesian Methods
The adoption of Bayesian methods in data science is driven by several advantages:
Incorporation of Prior Knowledge: Unlike frequentist statistics, which solely relies on observed data, Bayesian inference allows for the incorporation of prior knowledge or beliefs about the problem at hand. This makes Bayesian models more adaptable to different situations and often leads to more reliable results, especially in cases of limited data availability.
Uncertainty Quantification: Bayesian methods provide a natural way to quantify uncertainty by producing posterior distributions that reflect the uncertainty in model parameters or predictions. This information is crucial for decision-making and risk management, where understanding the reliability of predictions is as important as the predictions themselves.
Flexibility in Model Complexity: Bayesian frameworks accommodate complex models and hierarchical structures more seamlessly compared to traditional statistical methods. This flexibility is advantageous in modern data science applications, where datasets are often large, diverse, and high-dimensional.
Challenges and Considerations
While Bayesian methods offer many advantages, they also come with challenges:
Computational Intensity: Bayesian inference can be computationally demanding, especially for complex models or large datasets. Techniques like Markov Chain Monte Carlo (MCMC) and Variational Inference (VI) are commonly used to approximate posterior distributions efficiently.
Subjectivity in Prior Selection: The choice of prior distribution can influence the posterior results. Selecting informative priors based on domain knowledge is crucial but requires careful consideration to avoid biasing the results.
In conclusion, Bayesian methods represent a powerful and flexible approach to data analysis and inference in data science. By allowing the integration of prior knowledge with observed data, these methods provide deeper insights, more robust predictions, and better decision-making capabilities across various domains. As the field of data science continues to evolve, proficiency in Bayesian methods is increasingly valuable for professionals seeking to advance their careers and tackle complex analytical challenges. Whether you are embarking on a data science course, seeking data science certification, or looking to enhance your skills in Python for data science, understanding Bayesian methods will undoubtedly enrich your toolkit and expand your opportunities in the rapidly growing field of data science.
My name is Gour and I am a technical content writer for DataMites. DataMites provides Artificial Intelligence, Data science, Machine learning and Python Programming Courses.
Add Comment
Education Articles
1. Best Servicenow Training In Ameerpet | HyderabadAuthor: krishna
2. Mern Stack Online Training | Best Mern Stack Course
Author: Hari
3. Salesforce Crm Online Training | Salesforce Crm Training
Author: himaram
4. Oracle Fusion Financials Online Training At Rainbow Training Institute
Author: Rainbow Training Institute
5. Microsoft Fabric Certification Course | Microsoft Azure Fabric
Author: visualpath
6. Microsoft Dynamics Ax Training Online | Microsoft Ax Training
Author: Pravin
7. Aws Data Engineering Training Institute In Hyderabad
Author: SIVA
8. Top Skills Employers Seek In International Business Management Professionals
Author: jann
9. Unlock The Power Of Integration With Oracle Integration Cloud Training At Rainbow Training Institute
Author: Rainbow Training Institute
10. Emerging Trends In Salesforce Devops For 2025 And Beyond:
Author: Eshwar
11. How Digital Evidence Is Secured And Managed By Iso 27037 Consultants?
Author: Danis
12. Patient Reported Outcomes Clinical Research – A New Era 2024
Author: Aakash jha
13. Transform Your Home: 7 Must-have Dyslexia Support Resources Every Parent Should Get!
Author: Bradly Franklin
14. The Key To Your Pet’s Health And Happiness
Author: Sumit
15. How To Streamline Administrative Processes In Schools: A Comprehensive Guide
Author: Revamp