ALL >> Technology,-Gadget-and-Science >> View Article
Machine Learning For Data Quality And Cleansing In Data Services
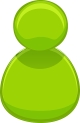
In today's data-driven world, the quality of data is paramount. Organizations rely on accurate, complete, and consistent data to make informed decisions, drive innovation, and maintain a competitive edge. However, ensuring data quality and cleansing vast amounts of data is a daunting task. This is where machine learning (ML) steps in, offering powerful tools and techniques to automate and enhance data quality and cleansing processes.
Understanding Data Quality
Data quality refers to the condition of data based on factors such as accuracy, completeness, reliability, relevance, and consistency. Poor data quality can lead to erroneous insights, misguided strategies, and financial losses. Key challenges in maintaining data quality include:
Data Inconsistency: Variations in data formats, units of measurement, or entry errors.
Data Incompleteness: Missing values or incomplete records.
Data Inaccuracy: Incorrect or outdated information.
Duplicate Data: Multiple records for the same entity.
The Role of Machine Learning in Data Quality
Machine learning, a subset of artificial intelligence, ...
... leverages algorithms to learn patterns from data and make decisions with minimal human intervention. When applied to data quality and cleansing, ML can:
Automate Data Cleaning: Identify and rectify errors, inconsistencies, and inaccuracies in data.
Enhance Data Integration: Merge and reconcile data from various sources.
Improve Data Enrichment: Augment data by filling in missing values and correcting inaccurate entries.
Detect Anomalies: Identify outliers and suspicious patterns that could indicate data quality issues.
Machine Learning Techniques for Data Cleansing
Data Imputation: Filling in missing values using statistical methods or ML algorithms like k-Nearest Neighbors (k-NN), regression, or deep learning models. For instance, k-NN can estimate a missing value based on the closest data points in the dataset.
Anomaly Detection: Using techniques like clustering, isolation forests, or neural networks to detect and handle outliers. Anomalies might indicate errors or rare events that need special attention.
Data Matching and Deduplication: Employing ML algorithms to identify and merge duplicate records. Techniques like fuzzy matching and probabilistic record linkage help in recognizing similar but non-identical records.
Text Normalization and Standardization: Applying natural language processing (NLP) techniques to clean and standardize text data. Tokenization, stemming, and lemmatization are common NLP tasks that improve text data quality.
Entity Resolution: Identifying and linking different records that refer to the same entity across multiple datasets. Machine learning models can be trained to recognize patterns and relationships between data points, facilitating accurate entity resolution.
Implementing ML for Data Quality: A Step-by-Step Guide
Data Profiling: Begin by analyzing the data to understand its structure, patterns, and quality issues. This step involves statistical analysis and visualization to identify anomalies, missing values, and inconsistencies.
Selecting ML Algorithms: Choose appropriate machine learning algorithms based on the data quality issues identified. For instance, use clustering for anomaly detection, regression for data imputation, and NLP for text normalization.
Model Training and Validation: Train the selected ML models on historical data with known quality issues. Validate the models using a separate dataset to ensure they generalize well to new data.
Automation and Integration: Integrate the trained models into data processing pipelines. Automate the data cleansing tasks and monitor the performance of the models regularly.
Continuous Improvement: Continuously monitor the data quality and the performance of the ML models. Update and retrain the models as needed to adapt to changing data patterns and quality issues.
Benefits of Using ML for Data Quality and Cleansing
Efficiency: Automates repetitive and time-consuming tasks, freeing up human resources for more strategic activities.
Accuracy: Reduces human error and improves the precision of data cleansing processes.
Scalability: Handles large volumes of data effectively, making it suitable for big data applications.
Adaptability: Learns and evolves with the data, improving over time with more data and feedback.
Conclusion
Machine learning is revolutionizing the way organizations handle data quality and cleansing. By automating and enhancing these processes, ML helps ensure that data remains accurate, complete, and reliable. This, in turn, enables organizations to make better decisions, drive innovation, and maintain a competitive edge in an increasingly data-centric world. As data continues to grow in volume and complexity, the role of machine learning in data quality and cleansing will become even more critical, paving the way for smarter and more efficient data management practices.
Add Comment
Technology, Gadget and Science Articles
1. How Predictive Analytics Can Help In Identifying High-quality LeadsAuthor: SalesMark Global
2. Trio3tech Laptop Screen Extender: The Ultimate Travel Companion For Productivity
Author: Triotech
3. Pharma Marketing: A Guide To Strategies, Benefits, And Challenges
Author: Jesvira
4. Endless Data's Ai And Fingerprint Recognition
Author: Endless Data
5. How To Choose The Best Food Delivery App Development Company To Create A Unique Platform
Author: Elite_m_commerce
6. How Ecommerce Seo Services In Dubai Can Boost Your Online Stores Visibility
Author: Markonik UAE
7. Unlocking Efficiency: Why Procurement Management Is Vital
Author: nagaraj
8. The Ultimate Guide To Using Vetro Power Waterproof Spray On Different Fabrics
Author: Your favourite outfits, accessories, and furniture
9. How Ecommerce Seo Services In Australia Can Boost Your Online Stores Visibility
Author: Markoni au
10. How Ecommerce Seo Services In India Can Boost Your Online Stores Visibility
Author: Markonik
11. Mastering The Art Of Storytelling With Ai
Author: Estefany Blanco
12. Laundry App Development - On-demand Cost & Features
Author: Deorwine Infotech
13. Lead The Way With Progressive Web Application Development
Author: Elite_m_commerce
14. Future-proof Your Business With Cloud Migration Solutions
Author: Hanry Brook
15. Augmented Reality: The Future Of Manufacturing
Author: Anastasia Green