ALL >> Education >> View Article
Classification Vs Clustering In Machine Learning: A Comprehensive Guide
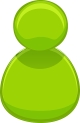
In the expansive realm of machine learning, two fundamental techniques stand out for their distinct purposes and methodologies: classification and clustering. Both play pivotal roles in data analysis, pattern recognition, and decision-making processes. Understanding the nuances between classification and clustering is crucial for aspiring data scientists and machine learning enthusiasts embarking on their Machine Learning Training Course journey. This comprehensive guide aims to demystify these techniques, providing insights into their differences, applications, and methodologies.
Understanding Classification
Classification is a supervised learning technique where the algorithm learns from labeled data to categorize input into predefined classes. It's akin to a teacher guiding students to identify objects based on their characteristics. During a Machine Learning Training Course, learners delve into various classification algorithms such as logistic regression, decision trees, support vector machines, and neural networks.
In classification, ...
... the model's goal is to accurately predict the class label for new data based on patterns learned from the training data. Applications of classification abound in diverse fields, including spam email detection, sentiment analysis, disease diagnosis, and image recognition.
Delving into Clustering
On the other hand, clustering is an unsupervised learning technique that groups similar data points into clusters based on their inherent characteristics. It's akin to organizing a diverse group of individuals into clusters based on their shared attributes without prior knowledge of specific labels. During a Machine Learning Training Course, students explore clustering algorithms like K-means, hierarchical clustering, and DBSCAN.
Clustering finds applications in customer segmentation, anomaly detection, recommendation systems, and genetic analysis. It's particularly useful in exploratory data analysis to uncover hidden patterns and structures within datasets.
Contrasting Methodologies
One key distinction between classification and clustering lies in their methodologies. In classification, the algorithm is trained on labeled data, with a clear objective to assign new data points to predefined classes. Conversely, clustering operates on unlabeled data, where the algorithm identifies similarities among data points to form clusters without explicit guidance on class labels.
During a Machine Learning Training Course, learners grasp the significance of data preprocessing, feature engineering, and model evaluation techniques tailored to each method. While classification focuses on optimizing predictive accuracy through metrics like accuracy, precision, recall, and F1-score, clustering evaluates cluster quality using measures such as silhouette score and Davies–Bouldin index.
Real-World Applications
Both classification and clustering techniques find widespread applications across various industries, showcasing their versatility and impact on decision-making processes. In a Machine Learning Training Course, students explore real-world case studies to understand how these techniques are employed to solve complex problems.
For instance, in e-commerce, classification algorithms are used for product recommendation and customer segmentation based on purchase history and preferences. Meanwhile, clustering techniques aid in identifying fraud detection patterns by grouping similar transactions for anomaly detection.
In conclusion, classification and clustering are two indispensable pillars of machine learning, each serving distinct purposes in data analysis and pattern recognition. Aspiring data scientists undergoing their Machine Learning Certification journey must grasp the differences between these techniques and their respective methodologies. Whether it's predicting customer churn or uncovering hidden patterns in genomic data, mastery of classification and clustering opens doors to a myriad of opportunities in the ever-evolving landscape of machine learning and data science.
Add Comment
Education Articles
1. Top Paying Careers In The Business Analytics SectorAuthor: Sarika
2. Study In Italy 2025: Free Consultation With Videsh Consultz
Author: Videsh
3. Data Science For Urban Air Quality Monitoring
Author: Gour
4. Tosca Training Institute In Hyderabad | Tosca Testing Online Training
Author: Susheelvisualpath
5. Deep Dive Into Fellowship In Reproductive Medicine
Author: Medline Academics
6. Devops Training In Hyderabad | Devops Training Institute In Ameerpet
Author: visualpath
7. Top Medical Universities In Turkey For Indian Students Pursuing Mbbs
Author: Mbbs Blog
8. Pursue Your Dream: Mbbs In Romania—affordable, World-class Education For Indian Students
Author: Mbbs Blog
9. Comprehensive Guide For Indian Mbbs Aspirants: Food And Hostel Facilities At Top Medical Universities In China
Author: Mbbs Blog
10. Exploring Non-traditional Pathways To Education: Online Courses And Beyond
Author: Briggate Educational Consultants
11. Best Seo Coaching & Online Classes For Learning Seo In 2024 | Captivate Designs
Author: Nicole McCullum
12. Canada Implements Temporary Cap On Study Permits To Address Housing Crisis
Author: luminarytimes
13. Why Switzerland Is The Top Destination For Studying Abroad: Insights From Our Education Fair
Author: Videsh
14. Bennett University: A Top Choice For Mba In Delhi
Author: Rohit Ridge
15. Azure Devops Training In Hyderabad | Azure Devsecops Training
Author: Renuka