ALL >> Computer-Programming >> View Article
Machine Learning Operations: A Comprehensive Guide To Demystifying
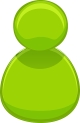
First of all,
With its ability to revolutionize decision-making processes and enable data-driven insights, machine learning (ML) models have become indispensable in many sectors. But creating a Machine Learning Models and putting it into production requires more complicated steps than just creating an algorithm. This is where MLOps, or Machine Learning Operations, are useful. The deployment, monitoring, and management of machine learning models in production environments can be made simpler and more automated with the help of a collection of procedures and tools called MLOps. We explore the depths of MLOps in this piece, offering a comprehensive guide to assist you in navigating this vital area of contemporary data science.
Step 1: Gathering and Preparing Data
Data is the cornerstone of any machine learning project. Strong data collecting techniques are the first step in MLOps, guaranteeing that pertinent and excellent data are accessible for model training. This include locating the sources of the data, removing noise from the data, and carrying out ...
... the necessary transformations to make the data compatible with operations that come after. Data engineers can efficiently preprocess data using tools like Pandas, TensorFlow Data Validation, and Apache Spark, which makes it possible to prepare datasets for model training.
Step 2: Model Development and Training: Data scientists start working on models after obtaining preprocessed data. Choosing the right algorithms, adjusting hyperparameters, and assessing model performance with methods like holdout validation and cross-validation are all part of this step. Teams can monitor changes in code, data, and configurations because to MLOps' emphasis on repeatability and version control. Collaborative model creation is made easier by platforms like MLflow and Git, which promote an experimental and iteration culture.
Step 3: Model Deployment: In MLOps, a model is put into production after it has been trained to a reasonable degree. This entails deploying the model on scalable infrastructure after packaging it with the required dependencies. Models can be regularly distributed across many environments thanks to the deployment process being made simpler by containerization technologies like Docker and orchestration tools like Kubernetes. Model updates may be smoothly sent out to production thanks to the deployment workflow automation provided by continuous integration/continuous deployment (CI/CD) pipelines.
Step 4: Upkeep and Observation:
The MLOps journey does not end with the deployment of a model into production; rather, it is just getting started. It takes constant observation to make sure the model functions as intended in practical situations. By identifying abnormalities and drifts in the distribution of data, monitoring tools like Grafana and Prometheus offer insights into the performance of the model. When the model's behavior deviates from the predicted norm, proactive action is made possible by automated notifications. Furthermore, to avoid model degradation over time and to stay up to date with changing data patterns, constant retraining of the model is required.
Step 5: Efficiency and Scalability:
As the need for machine learning applications increases, scalability in MLOps becomes increasingly important. Models can manage growing workloads by using horizontal scaling approaches, which divide processing among several nodes. Machine Learning Models deployment can be hosted and served on scalable infrastructure by cloud-based services such as Google Cloud AI Platform and Amazon SageMaker. In resource-constrained situations, optimization approaches like model quantization and pruning improve operational efficiency by lowering model size and inference latency.
Step 6: Governance and Compliance: Ensuring adherence to legal and ethical norms is crucial in regulated areas like healthcare and banking. Governance frameworks are incorporated into MLOps to mitigate concerns related to privacy, fairness, and bias in models. Sophisticated methods such as federated learning and differential privacy safeguard private user information while facilitating cooperative model training over dispersed datasets. Furthermore, model explainability tools foster accountability and transparency by assisting stakeholders in comprehending the reasoning behind model predictions.
In summary, MLOps, or machine learning operations, is a multidisciplinary methodology that connects machine learning research to practical implementation. Organizations can create powerful MLOps pipelines to expedite the development, deployment, and maintenance of machine learning models by following the detailed instructions provided in this article. Teams can offer dependable and scalable machine learning solutions that spur creativity and business value in the digital age by adopting MLOps techniques.
Our comprehensive MLOps services enable you to efficiently and effectively scale, deploy, monitor, and manage your Machine Learning Models, with an emphasis on model deployment. Together, you and our team of knowledgeable data scientists, engineers, and developers create and execute a personalized MLOps solution that addresses your unique requirements.
Add Comment
Computer Programming Articles
1. How Web Design Chicago Is Revolutionizing The User Experience For BusinessesAuthor: Tim Harrison
2. Best It Courses | Which Course In It Is Best?
Author: Kodestree
3. Uv Stabilizers Market Poised To Hit $1.90 Billion By 2032: Growth Trends & Insights
Author: Rutuja Kadam
4. The Ultimate Guide To Choosing The Best Cross-platform App Development Company For Your Business Success
Author: Siddharth
5. Stand-up Pouches Market To Be Worth $52.1 Billion By 2032
Author: Rutuja Kadam
6. Top Full Stack Development Services For Web And Mobile Apps In 2025
Author: jonathan
7. Revolutionize Your Online Presence With Cutting-edge Web Development!
Author: Hitvanshi
8. Top 10 Ibm I Service Providers Right Now
Author: Siddhant Saxena
9. How Mobile Applications Helps Local Businesses Grow?
Author: Ritesh Sharma
10. The Dynamic Landscape Of Advertising Agencies In Indonesia: A Hub For Creativity And Innovation
Author: milestone
11. 10 Advantages Of Building A Career In Full Stack Java Development In Bhopal
Author: Rohan Rajput
12. Top Mobile App Development Company In Canada: Comprehensive Solutions For Your Business
Author: iTechnolabs
13. Democratizing Development: The Rise Of Low-code And No-code Platforms
Author: Techeunoia International
14. Amazon Product Listing Services: Boost Your Sales With Optimized Listings
Author: rachelvandereg
15. Best Accounting Software 2025 In Zambia: Tips And Best Practices
Author: Doris oseR