ALL >> Education >> View Article
"data Lakes Vs. Data Warehouses: Choosing The Right Storage"
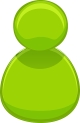
In the ever-evolving landscape of data management, businesses are faced with critical decisions on how to store and manage their data efficiently. Two prominent options, Data Lakes and Data Warehouses, have emerged as contenders for organizations seeking robust storage solutions. Understanding the distinctions between these two is crucial for making an informed choice tailored to specific business needs.
I. Introduction
In today's data-driven era, where information is a valuable asset, organizations are increasingly looking for advanced storage solutions. This article delves into the world of Data Lakes and Data Warehouses, exploring their characteristics, use cases, and key differences to help businesses make the right storage choice.
A. Definition of Data Lakes
Data Lakes are vast repositories that store raw and unstructured data. Unlike traditional databases, Data Lakes can hold diverse data types, including text, images, and videos. They serve as a central hub for storing large volumes of data, offering flexibility and scalability.
B. Definition of Data Warehouses
On the other hand, Data ...
... Warehouses are structured repositories designed for processing and analyzing structured data. They organize information in a tabular format, making it easy to query and derive insights. Data Warehouses are optimized for complex queries and are well-suited for business intelligence purposes.
C. Importance of Choosing the Right Storage
Choosing between Data Lakes and Data Warehouses is a critical decision that directly impacts an organization's data strategy, analytics capabilities, and overall efficiency. Let's explore each storage solution in detail to understand their nuances.
II. Understanding Data Lakes
A. Characteristics of Data Lakes
1. Flexibility: Data Lakes accommodate various data formats, allowing organizations to store diverse datasets without the need for pre-defined schemas.
2. Scalability: As data volumes grow, Data Lakes scale effortlessly, making them suitable for handling massive amounts of information.
3. Cost-Effective Storage: Storing raw data is cost-effective initially, as organizations only pay for storage without the need for extensive processing.
B. Use Cases of Data Lakes
1. Data Exploration and Discovery: Data Lakes are ideal for exploratory analysis, enabling data scientists to explore and discover insights without predefined structures.
2. Machine Learning and Advanced Analytics: The flexibility of Data Lakes supports machine learning models and advanced analytics that require diverse datasets.
C. Advantages and Challenges
1. Advantages: Flexibility, scalability, and cost-effective storage make Data Lakes suitable for organizations with diverse data needs.
2. Challenges: Managing metadata and ensuring data quality can be challenging in Data Lakes, requiring robust data governance practices.
III. Exploring Data Warehouses
A. Characteristics of Data Warehouses
1. Structured Data Storage: Data Warehouses organize data in a structured format, making it easy to query and analyze.
2. Optimized for Query Performance: Designed for efficient querying, Data Warehouses excel in processing structured data for business intelligence purposes.
B. Use Cases of Data Warehouses
1. Business Intelligence: Data Warehouses play a crucial role in generating insights for decision-making through ad-hoc queries and reporting.
2. Historical Data Analysis: Structured storage makes Data Warehouses suitable for analyzing historical trends and patterns.
C. Advantages and Challenges
1. Advantages: Optimized for performance, Data Warehouses are ideal for businesses requiring structured data analysis and reporting.
2. Challenges: Scalability challenges may arise with massive data volumes, and the cost of storage and processing can be higher compared to Data Lakes.
IV. Key Differences Between Data Lakes and Data Warehouses
A. Data Structure and Schema
1. Data Lakes: Support unstructured and raw data without predefined schemas.
2. Data Warehouses: Organize data in a structured format with predefined schemas.
B. Data Processing and Analysis
1. Data Lakes: Suited for exploratory analysis and machine learning models with raw and diverse data.
2. Data Warehouses: Optimized for query performance and business intelligence with structured data.
C. Storage Cost and Scalability
1. Data Lakes: Cost-effective storage initially; scalable to handle large volumes of diverse data.
2. Data Warehouses: Efficient for structured data analysis but may incur higher storage and processing costs.
V. Factors to Consider When Choosing Between Data Lakes and Data Warehouses
A. Type of Data
1. Nature of Data: Consider whether the data is structured or unstructured, and the need for flexibility in handling diverse datasets.
B. Analytical Requirements
1. Analytical Goals: Define the primary use of data, whether for exploratory analysis, business intelligence, or machine learning.
C. Budget Constraints
1. Cost Considerations: Evaluate the initial and long-term costs associated with storage, processing, and maintenance.
D. Scalability Needs
1. Data Growth: Assess the expected growth in data volume and choose a solution that scales accordingly.
VI. Real-World Examples
A. Case Study 1: Successful Implementation of Data Lake
In this case study, Company X leveraged a Data Lake to store and analyze diverse datasets, leading to enhanced insights and data-driven decision-making.
B. Case Study 2: Effective Use of Data Warehouse
Company Y implemented a Data Warehouse for structured data analysis, resulting in improved business intelligence and reporting capabilities.
VII. Best Practices for Implementation
A. Data Governance
1. Metadata Management: Implement robust metadata management practices to ensure data quality and traceability.
B. Integration with Existing Systems
1. Seamless Integration: Integrate Data Lakes or Data Warehouses with existing systems to ensure smooth data flow.
C. Security Measures
1. Data Security: Implement encryption and access controls to safeguard sensitive data.
VIII. Making the Decision
A. Evaluating Business Needs
1. Needs Assessment: Conduct a thorough assessment of business requirements and data characteristics.
B. Consulting with IT Experts
1. Expert Advice: Seek guidance from IT experts to understand the technical implications and long-term benefits.
C. Conducting a Cost-Benefit Analysis
1. Cost-Benefit Evaluation: Evaluate the costs and benefits associated with Data Lakes and Data Warehouses based on business priorities.
IX. Conclusion
In conclusion, the choice between Data Lakes and Data Warehouses depends on various factors, including data type, analytical goals, budget constraints, and scalability needs. Businesses must carefully assess their requirements and weigh the advantages and challenges of each storage solution before making a decision.
FAQs
1.Which is more cost-effective, Data Lakes, or Data Warehouses?The cost-effectiveness depends on factors such as data type, volume, and analytical requirements. Data Lakes offer cost-effective storage initially, while Data Warehouses may incur higher costs for structured data analysis.
2.Can Data Lakes handle structured data efficiently?While Data Lakes are designed for unstructured data, they can handle structured data with proper organization and metadata management.
3.What role does data governance play in the implementation of Data Lakes and Data Warehouses?Data governance is crucial for ensuring data quality, traceability, and security in both Data Lakes and Data Warehouses.
4.How do I determine the scalability needs for my organization?Assessing the expected growth in data volume and understanding future analytical goals will help determine scalability needs.
5.Where can I get more information on implementing Data Lakes or Data Warehouses for my business?For more detailed information and guidance, consult with IT experts and explore case studies of successful implementations in similar business contexts.
TO GET MORE INFORMATION VISIT OUR WEBSITE NOW https://ferilionlabs.com/
Add Comment
Education Articles
1. Advanced Diploma In Computer Applications: Full Form And Scope"Author: Kanika
2. Trends In Mobile Marketing To Watch Out For In 2025
Author: Guru360 Training Academy
3. How To Prepare For A Driving Crash Course In Manchester
Author: Michael Ware
4. What’s Included In A Guaranteed Pass Driving Course In Manchester?
Author: Michael Ware
5. Azure Data Engineering Certification | Azure Data Engineer
Author: gollakalyan
6. Mern Stack Course In Hyderabad | Mern Stack Online Training
Author: Hari
7. Top 22 Sre (site Reliability Engineer) Interview Questions & Answers 2025
Author: Vedanti
8. The Power Of Internships: Unlocking Career Opportunities While In College
Author: Patuck Gala College
9. The Ultimate Guide To Terraform Automation Online Training
Author: SIVA
10. Prompt Engineering Course | Prompt Engineering Ai Courses Online
Author: Anika Sharma
11. Top Foreign Study Consultancy In Warangal – Your Gateway To Global Education
Author: Johnwick
12. Microsoft Fabric Training In Hyderabad | Visualpath
Author: visualpath
13. Best Ssc Coaching In Delhi: A Complete Guide To Success
Author: Bst Competitive Classes
14. Unlocking The Potential With Oracle Integration Cloud Training
Author: Rainbow Training Institute
15. Every Thing You Should Know About Ras Exam 2025
Author: DrGenius Academy