ALL >> Education >> View Article
Strategies For Managing Missing Data In Analytics
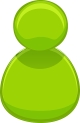
However, despite our best efforts, missing data is an inevitable challenge that analysts encounter. Whether it's due to human error, technical issues, or incomplete records, missing data can significantly skew analysis outcomes and compromise the reliability of insights derived from data analytics.
Fortunately, there are effective strategies and techniques to address missing data, ensuring that our analyses remain robust and trustworthy. Before delving into these strategies, it's crucial to recognize the impact of missing data on analytics projects and the need for adept management.
Missing data can manifest in various forms, including:
1. Missing Completely at Random (MCAR): Data is missing randomly, without any pattern or correlation to other variables.
2. Missing at Random (MAR): The probability of data being missing depends on other observed variables but not on the missing data itself.
3. Missing Not at Random (MNAR): The probability of data being missing depends on the missing data itself, leading to biased results.
Understanding the nature of missing data is pivotal in selecting ...
... appropriate strategies to handle it. Now, let's explore some effective approaches:
1. Data Imputation Techniques:
Data imputation involves estimating missing values based on available information. Techniques such as mean imputation, median imputation, and regression imputation can be employed to fill in missing values while preserving the integrity of the dataset.
2. Multiple Imputation:
Unlike single imputation methods, multiple imputation generates multiple plausible values for missing data, reflecting the uncertainty associated with the missing values. This approach enhances the accuracy of analysis results by accounting for variability in the imputed values.
3. Advanced Modeling Techniques:
Leveraging advanced modeling techniques such as machine learning algorithms, analysts can predict missing values based on the relationships observed within the dataset. This approach is particularly effective for handling complex datasets with numerous interdependencies.
4. Dropping Missing Data:
While it's essential to maximize data utilization, there are scenarios where dropping missing data may be a viable option, especially if the missingness is random and minimal. However, caution must be exercised to ensure that dropping data does not introduce bias into the analysis.
5. Sensitivity Analysis:
Conducting sensitivity analysis allows analysts to assess the robustness of their findings concerning missing data. By systematically varying assumptions regarding missing data patterns, analysts can gauge the stability of their conclusions under different scenarios.
Effective management of missing data is not only vital for ensuring the accuracy and reliability of analytics outcomes but also plays a pivotal role in data-driven decision-making processes. Organizations and professionals seeking to enhance their capabilities in data analytics must prioritize acquiring the necessary skills and knowledge to navigate this challenge effectively.
Enrolling in a reputable data analytics course equips individuals with the requisite expertise to proficiently handle missing data and other complexities inherent in analytics projects. A comprehensive data analytics training program provides participants with a deep understanding of statistical techniques, modeling methodologies, and practical tools for data manipulation and analysis.
Seeking certification from a recognized data analytics institute further validates one's proficiency in managing missing data and executing data analytics projects with precision. A data analyst training course that incorporates hands-on exercises and real-world case studies empowers participants to apply theoretical concepts in practical settings, thereby enhancing their problem-solving skills and analytical acumen.
While online resources offer convenience and flexibility, data analyst training certification programs conducted through offline classes facilitate interactive learning experiences and foster collaboration among participants. Engaging in offline classes allows learners to benefit from peer-to-peer interaction, instructor guidance, and immersive learning environments conducive to skill development.
Conclusion.
Managing missing data in analytics necessitates a multifaceted approach encompassing data imputation techniques, advanced modeling methodologies, and robust sensitivity analysis. By equipping oneself with the requisite skills through a comprehensive data analytics course and pursuing certification from a reputable institute, professionals can effectively navigate the challenges posed by missing data and deliver impactful insights through data analytics.
Add Comment
Education Articles
1. Can Ielts Academic Be Used For Immigration?Author: lily bloom
2. A Course On The Importance Of Upskilling At The College Level
Author: stem-xpert
3. Global Clinical Trials: Unveiling India’s Booming Market Outlook For 2025!
Author: Aakash jha
4. The Power Of Micro-influencers: Why They Matter More Than Ever
Author: dev
5. Digital Marketing Course In Dadar
Author: Sanchi
6. The Best Sre Course Online In India | Sre Training
Author: krishna
7. Top Google Cloud Ai Training In Chennai - Visualpath
Author: visualpath
8. Best Dynamics 365 Business Central Online Training - Hyderabad
Author: Susheel
9. The Best Data Engineering Course In Hyderabad - 2025
Author: naveen
10. Microsoft Dynamics 365 Course In Hyderabad | D365 Training
Author: Hari
11. Top Salesforce Marketing Cloud Training In Ameerpet
Author: Visualpath
12. Top Snowflake Course In Ameerpet | Snowflake Online Training
Author: Pravin
13. List Of Top Online Ma Colleges In India
Author: Study Jagat
14. Scrum Master Training In Bangalore | Scrum Master Classes
Author: visualpath
15. Mendix Online Training | Mendix Online Certification Course
Author: himaram