ALL >> Computers >> View Article
Big Data Architecture
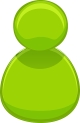
A big data architecture is designed to handle the ingestion, processing, and analysis of data that is too large or complex for traditional database systems. The threshold at which organizations enter into the big data realm differs, depending on the capabilities of the users and their tools. For some, it can mean hundreds of gigabytes of data, while for others it means hundreds of terabytes. As tools for working with big datasets advance, so does the meaning of big data. More and more, this term relates to the value you can extract from your data sets through advanced analytics, rather than strictly the size of the data, although in these cases they tend to be quite large.
Components of a big data architecture
Most big data architectures include some or all of the following components:
Data sources. All big data solutions start with one or more data sources. Examples include:
Application data stores, such as relational databases.
Static files produced by applications, such as web server log files.
Real-time data sources, such as IoT devices.
Data storage. Data for batch processing ...
... operations is typically stored in a distributed file store that can hold high volumes of large files in various formats. This kind of store is often called a data lake. Options for implementing this storage include Azure Data Lake Store or blob containers in Azure Storage.
Batch processing. Because the data sets are so large, often a big data solution must process data files using long-running batch jobs to filter, aggregate, and otherwise prepare the data for analysis. Usually these jobs involve reading source files, processing them, and writing the output to new files. Options include running U-SQL jobs in Azure Data Lake Analytics, using Hive, Pig, or custom Map/Reduce jobs in an HDInsight Hadoop cluster, or using Java, Scala, or Python programs in an HDInsight Spark cluster.
Real-time message ingestion. If the solution includes real-time sources, the architecture must include a way to capture and store real-time messages for stream processing. This might be a simple data store, where incoming messages are dropped into a folder for processing. However, many solutions need a message ingestion store to act as a buffer for messages, and to support scale-out processing, reliable delivery, and other message queuing semantics. This portion of a streaming architecture is often referred to as stream buffering. Options include Azure Event Hubs, Azure IoT Hub, and Kafka.
Stream processing. After capturing real-time messages, the solution must process them by filtering, aggregating, and otherwise preparing the data for analysis. The processed stream data is then written to an output sink. Azure Stream Analytics provides a managed stream processing service based on perpetually running SQL queries that operate on unbounded streams. You can also use open source Apache streaming technologies like Storm and Spark Streaming in an HDInsight cluster.
Analytical data store. Many big data solutions prepare data for analysis and then serve the processed data in a structured format that can be queried using analytical tools. The analytical data store used to serve these queries can be a Kimball-style relational data warehouse, as seen in most traditional business intelligence (BI) solutions. Alternatively, the data could be presented through a low-latency NoSQL technology such as HBase, or an interactive Hive database that provides a metadata abstraction over data files in the distributed data store. Azure Synapse Analytics provides a managed service for large-scale, cloud-based data warehousing. HDInsight supports Interactive Hive, HBase, and Spark SQL, which can also be used to serve data for analysis.
Analysis and reporting. The goal of most big data solutions is to provide insights into the data through analysis and reporting. To empower users to analyze the data, the architecture may include a data modeling layer, such as a multidimensional OLAP cube or tabular data model in Azure Analysis Services. It might also support self-service BI, using the modeling and visualization technologies in Microsoft Power BI or Microsoft Excel. Analysis and reporting can also take the form of interactive data exploration by data scientists or data analysts. For these scenarios, many Azure services support analytical notebooks, such as Jupyter, enabling these users to leverage their existing skills with Python or R. For large-scale data exploration, you can use Microsoft R Server, either standalone or with Spark.
Orchestration. Most big data solutions consist of repeated data processing operations, encapsulated in workflows, that transform source data, move data between multiple sources and sinks, load the processed data into an analytical data store, or push the results straight to a report or dashboard. To automate these workflows, you can use an orchestration technology such Azure Data Factory or Apache Oozie and Sqoop.
Benefits of Big Data Architecture
1. Parallel computing for high performance
To process large data sets quickly, big data architectures use parallel computing, in which multiprocessor servers perform numerous calculations at the same time. Sizable problems are broken up into smaller units which can be solved simultaneously.
2. Elastic scalability
Big Data architectures can be scaled horizontally, enabling the environment to be adjusted to the size of each workload. Big Data solutions are usually run in the cloud, where you only pay for the storage and computing resources you actually use.
3. Freedom of choice
The marketplace offers many solutions and platforms for use in Big Data architectures, such as Azure managed services, MongoDB Atlas, and Apache technologies. You can combine solutions to get the best fit for your various workloads, existing systems, and IT skill sets.
4. Interoperability with related systems
You can create integrated platforms across different types of workloads, leveraging Big Data architecture components for IoT processing and BI as well as analytics workflows.
Big Data Architecture Challenges
1. Security
Big data of the static variety is usually stored in a centralized data lake. Robust security is required to ensure your data stays protected from intrusion and theft. But secure access can be difficult to set up, as other applications need to consume the data as well.
2. Complexity
A Big Data architecture typically contains many interlocking moving parts. These include multiple data sources with separate data-ingestion components and numerous cross-component configuration settings to optimize performance. Building, testing, and troubleshooting Big Data processes are challenges that take high levels of knowledge and skill.
3. Evolving technologies
It’s important to choose the right solutions and components to meet the business objectives of your Big Data initiatives. This can be daunting, as many Big Data technologies, practices, and standards are relatively new and still in a process of evolution. Core Hadoop components such as Hive and Pig have attained a level of stability, but other technologies and services remain immature and are likely to change over time.
4. Specialized skill sets
Big Data APIs built on mainstream languages are gradually coming into use. Nevertheless, Big Data architectures and solutions do generally employ atypical, highly specialized languages and frameworks that impose a considerable learning curve for developers and data analysts alike.
Wisemonkeys(https://wisemonkeys.info/), is an LMS platform with unique features like “Ask a question” and get it answers by experts within no time . It’s a mass initiative to spread knowledge worldwide, truly believe in “When we know knowledge is free then why not spread it.” From various sectors to industries, write your heart out and get noticed.
Explore Wisemonkeys now.(https://me.wisemonkeys.info/login)
For the Monkeys | By the Monkeys.
Add Comment
Computers Articles
1. 10 Dropshipping Shopify Apps For Shopify Stores 2025Author: Elightwalk Technology
2. How To Write A Great Creative Ux Brief For A Design Consultancy
Author: goodcoders
3. How To Market Your Gojek Clone App Effectively
Author: simonharris
4. Scrape Restaurant Guru Review Data: Unlock Actionable Insights
Author: DataZivot
5. How To Make A Rental Search App To Solve Tenants’ Problems?
Author: goodcoders
6. Is The Is200ehpag1d Exciter Gate Pulse Amplifier Board The Ideal Choice For Your Power System Needs?
Author: Alex Zilk
7. Document Management System For Clinical Trials
Author: Giselle Bates
8. Vupico Sdp
Author: Lorenzoe Taala
9. An Extensive Guide On E-commerce Application Development
Author: goodcoders
10. Why Do Your Businesses Need Vehicle Rental Management Software In 2025?
Author: RentAAA
11. Exploring Bigpond A Reliable Choice For Your Internet Needs
Author: james smith
12. Elevate Your Online Presence With Leading Digital Marketing Solutions In Surat
Author: sassy infotech
13. How To Power Several Appliances Together Safely?
Author: Jennifer Truong
14. Web Scraping Zomato Restaurant Customer Reviews
Author: DataZivot
15. Why Every Coworking Space Needs Management Software To Thrive
Author: RentAAA